Open Access
ARTICLE
Malicious Traffic Compression and Classification Technique for Secure Internet of Things
1 Department of Future Convergence Technology Engineering, Sungshin Women’s University, Seoul, 02844, Korea
2 Department of Convergence Security Engineering, Sungshin Women’s University, Seoul, 02844, Korea
* Corresponding Author: Il-Gu Lee. Email:
(This article belongs to the Special Issue: Innovative Security for the Next Generation Mobile Communication and Internet Systems)
Computers, Materials & Continua 2023, 76(3), 3465-3482. https://doi.org/10.32604/cmc.2023.041196
Received 14 April 2023; Accepted 07 July 2023; Issue published 08 October 2023
Abstract
With the introduction of 5G technology, the application of Internet of Things (IoT) devices is expanding to various industrial fields. However, introducing a robust, lightweight, low-cost, and low-power security solution to the IoT environment is challenging. Therefore, this study proposes two methods using a data compression technique to detect malicious traffic efficiently and accurately for a secure IoT environment. The first method, compressed sensing and learning (CSL), compresses an event log in a bitmap format to quickly detect attacks. Then, the attack log is detected using a machine-learning classification model. The second method, precise re-learning after CSL (Ra-CSL), comprises a two-step training. It uses CSL as the 1st step analyzer, and the 2nd step analyzer is applied using the original dataset for a log that is detected as an attack in the 1st step analyzer. In the experiment, the bitmap rule was set based on the boundary value, which was 99.6% true positive on average for the attack and benign data found by analyzing the training data. Experimental results showed that the CSL was effective in reducing the training and detection time, and Ra-CSL was effective in increasing the detection rate. According to the experimental results, the data compression technique reduced the memory size by up to 20% and the training and detection times by 67% when compared with the conventional technique. In addition, the proposed technique improves the detection accuracy; the Naive Bayes model with the highest performance showed a detection rate of approximately 99%.Keywords
Cite This Article
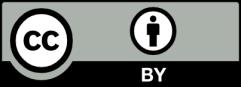