Open Access
ARTICLE
Traffic Flow Prediction with Heterogenous Data Using a Hybrid CNN-LSTM Model
1 Departement of Computer Science and Information Engineering, Asia University, Taichung, Taiwan
2 Departement of Information Technology, Universitas Muhammadiyah Yogyakarta, Yogyakarta, Indonesia
* Corresponding Author: Chayadi Oktomy Noto Susanto. Email:
Computers, Materials & Continua 2023, 76(3), 3097-3112. https://doi.org/10.32604/cmc.2023.040914
Received 04 April 2023; Accepted 07 June 2023; Issue published 08 October 2023
Abstract
Predicting traffic flow is a crucial component of an intelligent transportation system. Precisely monitoring and predicting traffic flow remains a challenging endeavor. However, existing methods for predicting traffic flow do not incorporate various external factors or consider the spatiotemporal correlation between spatially adjacent nodes, resulting in the loss of essential information and lower forecast performance. On the other hand, the availability of spatiotemporal data is limited. This research offers alternative spatiotemporal data with three specific features as input, vehicle type (5 types), holidays (3 types), and weather (10 conditions). In this study, the proposed model combines the advantages of the capability of convolutional (CNN) layers to extract valuable information and learn the internal representation of time-series data that can be interpreted as an image, as well as the efficiency of long short-term memory (LSTM) layers for identifying short-term and long-term dependencies. Our approach may utilize the heterogeneous spatiotemporal correlation features of the traffic flow dataset to deliver better performance traffic flow prediction than existing deep learning models. The research findings show that adding spatiotemporal feature data increases the forecast’s performance; weather by 25.85%, vehicle type by 23.70%, and holiday by 14.02%.Keywords
Cite This Article
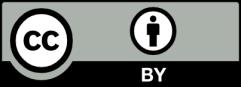
This work is licensed under a Creative Commons Attribution 4.0 International License , which permits unrestricted use, distribution, and reproduction in any medium, provided the original work is properly cited.