Open Access
ARTICLE
Intelligent Traffic Surveillance through Multi-Label Semantic Segmentation and Filter-Based Tracking
1 Department of Creative Technologies, Air University, Islamabad, 44000, Pakistan
2 Department of Information Systems, College of Computer and Information Sciences, Princess Nourah bint Abdulrahman University, P.O. Box 84428, Riyadh, 11671, Saudi Arabia
3 Information Systems Department, Umm Al-Qura University, Makkah, Saudi Arabia
4 Department of Computer Science, College of Sciences and Humanities in Aflaj, Prince Sattam Bin Abdulaziz University, Al-Kharj, Saudi Arabia
5 Department of Computer Engineering, Tech University of Korea, Siheung-si, Gyeonggi-do, 15073, Korea
* Corresponding Author: Jeongmin Park. Email:
Computers, Materials & Continua 2023, 76(3), 3707-3725. https://doi.org/10.32604/cmc.2023.040738
Received 29 March 2023; Accepted 04 July 2023; Issue published 08 October 2023
Abstract
Road congestion, air pollution, and accident rates have all increased as a result of rising traffic density and worldwide population growth. Over the past ten years, the total number of automobiles has increased significantly over the world. In this paper, a novel method for intelligent traffic surveillance is presented. The proposed model is based on multilabel semantic segmentation using a random forest classifier which classifies the images into five classes. To improve the results, mean-shift clustering was applied to the segmented images. Afterward, the pixels given the label for the vehicle were extracted and blob detection was applied to mark each vehicle. For the validation of each detection, a vehicle verification method based on the structural similarity index is proposed. The tracking of vehicles across the image frames is done using the Identifier (ID) assignment technique and particle filter. Also, vehicle counting in each frame along with trajectory estimation was done for each object. Our proposed system demonstrated a remarkable vehicle detection rate of 0.83 over Vehicle Aerial Imaging from Drone (VAID), 0.86 over AU-AIR, and 0.75 over the Unmanned Aerial Vehicle Benchmark Object Detection and Tracking (UAVDT) dataset during the experimental evaluation. The proposed system can be used for several purposes, such as vehicle identification in traffic, traffic density estimation at intersections, and traffic congestion sensing on a road.Keywords
Cite This Article
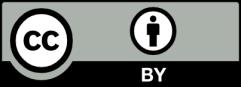
This work is licensed under a Creative Commons Attribution 4.0 International License , which permits unrestricted use, distribution, and reproduction in any medium, provided the original work is properly cited.