Open Access
ARTICLE
A Hybrid Deep Learning Approach to Classify the Plant Leaf Species
1 Department of CS&SE, Islamic International University, Islamabad, 44000, Pakistan
2 Department of IT Services, University of Okara, Okara, 56310, Pakistan
3 Faculty of Science and Arts Belqarn, University of Bisha, Sabtul Alaya, 61985, Saudi Arabia
4 Department of CS, University of Okara, Okara, 56310, Pakistan
5 Department of Computer Science & Engineering, University of Engineering & Technology Lahore, Narowal, Campus, Narowal, 51601, Pakistan
6 Electrical Engineering Department, College of Engineering, King Khalid University, Abha, 61421, Saudi Arabia
7 Research Center for Advanced Materials Sciences (RCAMS), King Khalid University, Abha, Saudi Arabia
* Corresponding Author: Mubbashar Saddique. Email:
Computers, Materials & Continua 2023, 76(3), 3897-3920. https://doi.org/10.32604/cmc.2023.040356
Received 15 March 2023; Accepted 30 May 2023; Issue published 08 October 2023
Abstract
Many plant species have a startling degree of morphological similarity, making it difficult to split and categorize them reliably. Unknown plant species can be challenging to classify and segment using deep learning. While using deep learning architectures has helped improve classification accuracy, the resulting models often need to be more flexible and require a large dataset to train. For the sake of taxonomy, this research proposes a hybrid method for categorizing guava, potato, and java plum leaves. Two new approaches are used to form the hybrid model suggested here. The guava, potato, and java plum plant species have been successfully segmented using the first model built on the MobileNetV2-UNET architecture. As a second model, we use a Plant Species Detection Stacking Ensemble Deep Learning Model (PSD-SE-DLM) to identify potatoes, java plums, and guava. The proposed models were trained using data collected in Punjab, Pakistan, consisting of images of healthy and sick leaves from guava, java plum, and potatoes. These datasets are known as PLSD and PLSSD. Accuracy levels of 99.84% and 96.38% were achieved for the suggested PSD-SE-DLM and MobileNetV2-UNET models, respectively.Keywords
Cite This Article
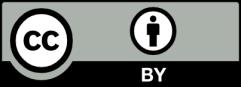
This work is licensed under a Creative Commons Attribution 4.0 International License , which permits unrestricted use, distribution, and reproduction in any medium, provided the original work is properly cited.