Open Access
REVIEW
A Survey on the Role of Complex Networks in IoT and Brain Communication
1 Cybersecurity Department, Faculty of Computing, Engineering and Science, University of South Wales, Wales, UK
2 Department of Computer Science, Faculty of Computing and Information Technology (FCIT), King Abdulaziz University, Jeddah, 21589, Saudi Arabia
3 Computer Science Department, Umm Al-Qura University, Makkah, 21955, Saudi Arabia
4 Department of Computer Science & IT, University of Lakki Marwat, Lakki Marwat, 28420, KPK, Pakistan
5 Department of Computer Engineering, Chungnam National University, Daejeon, 34134, Republic of Korea
* Corresponding Author: Muhammad Shuaib Qureshi. Email:
(This article belongs to the Special Issue: Applications of Digital Twins in Intelligent Healthcare Systems)
Computers, Materials & Continua 2023, 76(3), 2573-2595. https://doi.org/10.32604/cmc.2023.040184
Received 07 March 2023; Accepted 18 July 2023; Issue published 08 October 2023
Abstract
Complex networks on the Internet of Things (IoT) and brain communication are the main focus of this paper. The benefits of complex networks may be applicable in the future research directions of 6G, photonic, IoT, brain, etc., communication technologies. Heavy data traffic, huge capacity, minimal level of dynamic latency, etc. are some of the future requirements in 5G+ and 6G communication systems. In emerging communication, technologies such as 5G+/6G-based photonic sensor communication and complex networks play an important role in improving future requirements of IoT and brain communication. In this paper, the state of the complex system considered as a complex network (the connection between the brain cells, neurons, etc.) needs measurement for analyzing the functions of the neurons during brain communication. Here, we measure the state of the complex system through observability. Using 5G+/6G-based photonic sensor nodes, finding observability influenced by the concept of contraction provides the stability of neurons. When IoT or any sensors fail to measure the state of the connectivity in the 5G+ or 6G communication due to external noise and attacks, some information about the sensor nodes during the communication will be lost. Similarly, neurons considered sing the complex networks concept neuron sensors in the brain lose communication and connections. Therefore, affected sensor nodes in a contraction are equivalent to compensate for maintaining stability conditions. In this compensation, loss of observability depends on the contraction size which is a key factor for employing a complex network. To analyze the observability recovery, we can use a contraction detection algorithm with complex network properties. Our survey paper shows that contraction size will allow us to improve the performance of brain communication, stability of neurons, etc., through the clustering coefficient considered in the contraction detection algorithm. In addition, we discuss the scalability of IoT communication using 5G+/6G-based photonic technology.Keywords
This paper introduces IoT and brain communication using the complex network’s concept and 5PSC (5G+/6G-based photonic sensor communication) technology. Although photonic elements are not in the neurons or brain cells explicitly, photonic sensors technology enhances the existing approaches used for analyzing neuron interactions. Thought creations and feelings which include pain and sensation depend on the communication of neuron sensors. When these neurons lose the energy gained through the protein molecules, talk between the neurons is disconnected. Hence, the continuous immune system becomes weak without regular communication and transmission of any neurons. Understanding and controlling the neuron activities in the normal and abnormal conditions of the body allow researchers to improve the accuracy of the solutions considered in brain diseases.
A critical open question in brain communication and IoT networks opens the scalability, which is the limitation of computer science as well as neuroscience. The scalability of IoT and neurons will be an interesting problem because IoT sensors are visible to analyze the scalability. Although the scalability of neuron sensors may have the same concept, they are invisible to our naked eyes. Analyzing the scalability of neurons within a cubic micrometer will allow us to understand the interaction and communication details of neurons. These details certainly improve brain communication and control the immune system when infections and pains are within the body. Although this survey paper not only focuses on scalability but also other potential issues such as secure medical systems. Regarding brain communication, existing neurons’ coordinated activities pass on the necessary information to newly born neurons. Dynamics research in complex networks offers many methods and approaches [1] for improving the controllability of dynamic systems where communication functions provide efficient connectivity links between the IoT nodes. Emergence is an essential characteristic of complex systems and networks [2]. In complex network analysis, strong and weak emergence can be considered for investigating the performance of network communication.
According to recent studies, by 2025 humanity will have created about 163 zettabytes of information [3]. Several brain diseases, such as neurological diseases and psychological disorders appear through the defects of brain communication. It means that necessary data and information should be within each time frame or period of communication. Here, time-varying issues are a very important factor for analyzing all defects that occurred in the connecting links. In brain communication, we draw particular focus to the topic of complex networks which deal with multi-slice/layer community detection. Also, the connection between the neurons depends on the complex networks where the role in characterizing time-varying connectivity can be an interesting issue analyzed through the multi-slice/layer community detection.
Defected links during brain communication do not send the correct activities to the receiving nodes of the neurons. The groups of neurons handle the specific type of information considered characteristic of brain functions. In the brain, all functions such as perceptions of received senses, feelings of actions, adaptive behaviors during continuous problems, etc.
Groups of neurons interconnected within which form the specific brain function define the cluster. Mathematically, the locations of these neurons create multi-dimension sparse matrices. In this survey paper, a combination of the community detection algorithm and manifold techniques will allow us to create a new concept and tool, which minimizes the complexity and energy consumption during the testing of brain communication.
The scalability of IoT can be comparable with the complex structure of neurons located within the brain. When the scalability of IoT nodes increases, the space of the brain will not be enough to accommodate all data and information handled by the IoT nodes. In the future, miniaturized IoT nodes may be developed to analyze brain functions, actions of thoughts, etc.
The role of complex networks in IoT and brain communication will enhance the strong detecting capacity for spiking solutions to brain diseases and defective neurons. Groups of neural units are used for controlling the specific feeling, pain, disease, etc., but when two groups are interconnected with other neural units, controlling communication activities will be difficult. In this case, the modularity maximization approach can be used in the community detection algorithm which allows us to enhance the functionalities of the brain’s complex network. Here, brain complex graphs can be constructed from a group of neural units considered neuroimaging data.
The paper is organized as follows. In Section 2, we describe the evolution of 5G communication which focuses on neuron and photonic concepts considered with sensors. Section 3 defines the key enabling technologies for 5PSC developed from the complex network theories and algorithms. Then, in Section 4 we demonstrate the role of the complex networks in IoT communication dealing with sensors that create the connectivity in brain communication with the available photonic energy and the concepts of neuroscience. The role of the complex network in brain communication will be considered with many challenges in Section 5. To understand the literature of this research paper, recent advances, and related works are summarized in Section 6. Finally, the main conclusions are given in Section 7.
2 Evolution of 5G Communication
Complex networks in IoT and brain communication are the new evolving techniques for improving the features of signal exchanging capacities between the sensors considered as neurons. Although 6G evolved from the features of 5G, the key expected characteristics of 6G depend on these requirements. They are spatial multiplexing, higher spectral efficiency (100 bps/Hz), ultra-high wireless security, ultra-reliability, ultra-low-power consumptions, and massively connected complex networks. Microwave communications also support 5G+ designs in which many recent advances (design of THz electronic, photonic, and hybrid electronic–photonic technologies) are encouraging. In the 5G+ and 6G, the evolution of a Terahertz (THz) radio spectrum may influence hybrid electronic–photonic transceivers [4].
2.1 Emerging Technologies of 5G and Beyond
The users’ requirements provide improvement and enhancement with the emerging technology. When the number of users is increasing, accessing techniques include dynamic orthogonal multiple access (D-OMA) and massive multiple access can enhance the accessing facilities through 5G+ and 6G [5–7].
In above Table 1, the accuracy of latency is still challenging with specific applications. In controllability, an acronym of RAMS means Reliability, Availability, Maintainability, and Scalability should be considered to control the 5G+/6G from the security. Computation of dynamic bandwidth should be managed efficiently with natural energy sources such as solar power which improves the EE. Despite many ways of achieving SE, dynamically managing and sharing the spectrum will increase SE. As far as SDN is concerned, 5G+/6G will be deploying SDN-based technologies to improve the demands and challenges of future applications. Table 2 shows the emerging 5G technologies which provide some smart approaches with the frontal haul (FH) and backhaul (BH), and challenges [8].
Although 5G+ expects to solve many challenges, emerging 5G technologies allow us to note quick opportunities, challenges, and solutions for illustrating some applications.
Opportunities: Although many hottest trends in communication technology use opportunities to transform the future using dynamic solutions, few intelligent approaches increase the opportunities for improving the applications of communication. Ultra-compact devices emerged with 5G communication, and network technologies can handle the multi-polarization environment and multiband. Here, many opportunities can be raised to improve the research of dynamic spectrum; they are such as hybrid (solar and wireless power) and energy harvesting micro electromechanical Systems (EH-MEMS). Also, these devices can function in a wide dynamic range with high-frequency operations.
Challenges: According to future applications such as driverless vehicles depend on many challenges such as intelligent-based priority and dynamic solutions. These challenges have to be considered with the emerging 5G communication technologies. Although this article introduces some challenges, we will be facing many evolving problems such as security and energy efficiency with a growing number of users and all types of communication devices.
Solutions: Tactile Internet requires some improvements in the design and solutions which depend on a significant reduction in latency. Although the concepts of the Tactile Internet are defined, future challenges of design and solutions depend on the emerging technologies of 5G. It allows remote users to improve their real-time control through ultra-reliable and ultra-responsive network connectivity. Despite many solutions from the upper layers, some hardware solutions depend on micro electromechanical systems (MEMS). In the future, the Internet, a smart solution for minimizing energy costs depends on energy harvesting micro electromechanical Systems (EH-MEMS), and radio frequencies micro electromechanical systems (RF-MEMS) intend to use in the 5G applications and beyond. Especially wireless communications and applications above 100 GHz will open many opportunities and challenges for 6G and beyond. For instance, building bridges toward the 6G-enabled wireless smart society and ecosystem will face many challenges in the 6G applications. In the complex network, airplane-aided integrated networking for 6G wireless communication, reconfigurable intelligent surfaces towards 6G, and developing 6G with MIMO are the specific challenges. Thus, the six key enablers are elaborated for machine-type communication considered in 6G [9–14].
2.2 Complex Networks in 5G Communication
This survey paper focuses on exploring IoT and brain communication influenced by complex networks. Although 5G gains limited benefits in employing complex networks, 5G+, and 6G will certainly employ complex networks for enhancing features of IoT scalability problems. One of the expected characteristics of 6G is massively connected complex networks which allow researchers to investigate the scalability of IoT and brain communication. According to [15], complex networks allow us to learn the fast consensus clustering algorithm which enhances the testing facilities of complex networks.
2.3 Photonic Technologies for 5G and Beyond
Accurate and fast photonic sensors with neuron information considered multi-sensor data fusion are the sense organs of digital medical developments. These optical and light-based technologies, therefore, feed new Artificial Intelligence (AI) algorithms to enable autonomous systems of reading and understanding the neurons’ communication, which will be future breakthroughs in medicine and healthcare applications. According to [16], photonic technology increases the pumping intensity when the biological medium of the DsRed2 fluorescent protein is in femtosecond pumping which involves two-photon absorption of femtosecond pulses. The study of femtosecond pulses and radiation introduces a new tool for developing medical applications. High intensity and wide optical spectrum considered properties of femtosecond enhance the facilities to improve brain communication.
2.4 Photonic Sensor Communication
A plasmonic-based compact and broadband polarizer, capable of producing a single polarized ray concurrently around the two communication windows of 1.31 and 1.55 μm, based on hexagonal lattice photonic crystal fiber is proposed. In an optical waveguide, photonic technology could provide some benefits to improve the long interconnect distances when dielectric and plasmonic waveguiding is in the designs of sensor communications. THz band wireless communication and wireless THz nano-applications depend on plasmonic technology.
Although there are many roles available in different applications, communication including 5PSC and brain communication will be the focus of this paper. Creating photonic integrated circuits is one of the photonics’ roles and allows us to improve the sensor communication concepts considered in brain communication. Photonic sensing increases the monitoring facilities such as the level of oxygen in each neuron, which provides neuron communication and early warning of brain diseases. Affordable medical treatment, assessment, and practical implementation of precision medicine will be possible with a photonics-based real-time diagnostics system. Terahertz photonic chips will improve communication and intelligence when a complex network is in the diagnostics system. Photonics communication technologies are the low-cost optical system for improving our lifestyle transformation which includes modern medical facilities, economy, transportation, etc.
1) Types of Photonics
Microwave photonics is one of the emerging types in modern technology involved with optics and future applications. Types of photonics depend on the properties of photons and external conditions such as temperature and light.
Table 3 shows the comparison of the different types, and numbers of components that comprise microelectronics, photonics, and silicon photonics [17].
Some types of photons are shown in Table 3, but silicon photonics allows us to investigate brain communication and neuron reactions.
2) Light and Photonic Sensors
There are significant approaches for integrating light-based developments in life sciences and health. In brain communication, the increase of brain-related diseases as well as diagnosing costs of the brain-related healthcare system is inevitable with the human population and polluted environment which affects the breathing air, eating food, etc.
A microelectronic pill is a multi-channel photonic sensor used for remote biomedical measurements using micro technology (Micro Tech). This has been developed for the internal study and detection of diseases and abnormalities in the gastrointestinal tract.
The effectiveness of treatments within the brain will be a big breakthrough in medical science focused on light-based theories. Here, solutions depend on light-based developments integrated with both electronics and photonics systems. Sensor communication involved with photonic technology enhances the impact of solutions for detecting and resolving damaged neurons in the brain.
Photonic sensors are the source of many devices that could be used in the medical system for improving healthcare costs. All brain-related diseases, symptoms of infections, and other pains will depend on the quick diagnostic system with early warning. Scientists have already developed wearable photonic devices for age-related diseases and photonics-based real-time diagnostics for identifying the status of the disease. Mobile and wearable photonic devices integrated with advanced biosensors provide extra healthcare for monitoring the wearers’ medical conditions and wellness. Further, photonics-based systems not only reduce overall healthcare and medical costs but also enhance medical facilities such as quick treatment responses, precision medicine, etc.
3 Key Enabling Technologies for 5PSC
Photonics, the manipulation of natural light sources at the nanoscale, is a key enabling technology that evolved with 5G+/6G which supports the impact on health and energy applications. In most cases, photonics still relies on materials that contain solar elements, and they depend on the fabrication methods inherited from miniaturized and environmentally unfriendly processes. As a diatomic molecule, slabs of photonics show the dimensions as high. Communication between the neurons precipitated by the impact of diatomic molecules is relying on intracellular bio-mineralization mechanisms [18].
The scalability of IoT using complex networks can be useful for analyzing the scalability of neurons and comparing the measurements related to the scalability. Communication in the brain involves many neurons or nodes of complex networks where scalability depends on the active nodes. The scalability of IoT can grow with the increasing number of applications and problems but it should be limited to the cubic meter. Here, scalability is proportional to the number of IoT sensors or devices allocated without any unnecessary interferences between the sensors. The following examples show the complex network issues in brain communication when neurons or active nodes are interacting with each other’s messages.
The complex network architecture of the human brain contains neurons and visible and invisible links as shown in Table 4. Analyzing complex networks has become a feature of increasing interest to the neuroscientific community because true networks and observed configurations are different. The observed network configurations may have missing links or spurious problems when the human brain is not functioning properly, largely because of its potential to illuminate human cognition, its variation over development and aging, and its alteration in disease or injury. Sensors in complex networks can be considered with photonic sensor technology. Having defined integrated photonics, a photonic integrated circuit of the photonic communication system supports brain communication which allows us to build an efficient healthcare system that includes the monitoring system of brain cancer. This integrated circuit will be improving the basic requirements of 5G+ and 6G developments when it involves terahertz communication and emerging technology.
3.2 Challenges of Complex Networks
Machine learning (ML) will be one of the future challenges when communication service providers employ complex networks for improving 5G+ and 6G requirements. In IoT sensor and brain communication, complex networks deal with the characteristic of artificial controlling and decision-making for measuring the movements of neurons.
Convolutional Neural Network in Complex Network enhances and supports the neurons, brain, and quantum communication. The artificial neural network (ANN) structure supports holding many layers for Quantum Machine Learning (QML) which allows us to set the new features of 6G Communication Networks [19]. Complex networks enhance the users’ requirements of 6G through the ambitious deep, wide, and complex ANN. Optimum fitting and quantum computing (QC) will be possible with the complex ANN but holding a balance between underfitting and overfitting is one of the issues in this approach. Novel QC-assisted and QML-based frameworks for 6G communication networks enhance the issues of 6G visions such as the massively connected complex network. According to [19–25], use cases and technologies of 6G allow researchers to verify the improvements of users’ requirements of 6G and analyze the vision of 6G wireless systems considered with applications of vehicular network communication, trends involved with AI, emerging technologies, and open research problems such as battery life.
According to [26,27], the waveguide network supports improving features of the modern architecture for photonic networks-on-chip. Here, the photonic network influenced by the light rays and colors can be analyzed from the source using the complex networks theory. The factors affecting the prediction of energy consumption and IoT modeling in complex networks are studied in [28]. Also, optimization of energy consumption is considered with data mining and other ML algorithms.
The ML for optical wireless and IoT communication can be considered in brain communication. Learning-based networking systems will be the key requirement in future 6G communication networks. The ever-increasing complex network structure and requirements demand artificial controlling and decision-making in challenging environments.
4 Role of the Complex Networks in IoT Communication
We reveal a type of sensors and IoT devices considered with photonic technology for improving brain communications. Further, we reveal a variety of sensor connections and dynamics, which follows complex networks in 5PSC. Regarding brain communication, the role of complex networks is to vary the inner topology of the neurons from specific clusters to different clusters. We find that an increment in the specific connectivity of sensor networks provides the enhancement of neuron connectivity in brain communication. The connectivity of IoT devices is also increasing with the scalability of IoT users, which includes the sensors. Cisco estimates that by 2020, more than 50 billion objects will be socially connected with the help of IoT and cloud technology. The role of complex networks in IoT communication will be evolving with energy-efficient technology. It means that the complexity of the systems will have many low-complex algorithms, protocols, and functions. To implement brain communication, the role of complex networks with IoT based on the photonic sensor would be advantageous.
4.1 Enhancement of Connectivity in Brain Communication
The inner topology of the neuron connections provides the specific function within the brain for understanding the internal and external reactions. In the sensor connections, the proposed research model is enhancing how our brain processes real information through the five senses. Regarding visual information, connectivity should be active for passing on information from the eye to downstream brain regions that guide behavior through the neurons. Knowledge of these five senses and behavior is essential to understanding how our brain signals and sensory information guides our actions and decisions [29,30].
4.2 Energy Management for Enhancing Brain Functions
We need to improve our immune system which is the main objective of energy management for enhancing brain functions controlled by the neurons. Although brain communications depend on the energy contained in the neurons, energy stored within the photonic elements may not be transferred to the neurons or brain cells directly. Energy management within the photonic sensors’ technology will enhance the energy-transferring process and the approaches used for analyzing neurons’ energy transactions. With the available energy, brain communication functions, and photonic sensor communications that support the monitoring facilities such as the level of oxygen in each neuron can be considered in energy-efficient medical applications. Function considered with the complex network algorithms will minimize the energy cost and enhances the EE. Energy performance and EE of neuron communications and early warnings which allow future devices to analyze brain diseases can be done with photonic technology. The devices with photonic integrated circuit and the photonic communication system as shown in Fig. 1 will be energy-efficient and reliable for analyzing brain communication issues.
Figure 1: A photonic integrated circuit of a photonic communication system
With the complex network theory and energy-efficient algorithms, this integrated circuit will be enhancing the accuracy of medical devices and administrative monitoring. Neuromorphic photonics and neurons are evolving technology for developing medical communication devices with AI and EE optimized through complex networks. To analyze the observability and brain communication performance, a contraction detection algorithm can be considered with complex network properties and 5PCS.
The IoT sensors are nodes used in complex networks which support measuring the performance of IoT communications and connections. Using the IoT and AI-based complex networks extracts the neuron communications from the brain as brain communication to external systems.
5 Role of the Complex Networks in Brain Communication
The network architecture of the human brain depends on the active neurons which exchange neuro communications through the complex network. The neuroscientific community expects to analyze brain functions involved with human cognition, its variation over aging, and its alteration in disease or injury. The role of complex networks in brain functions and communications will depend on the analysis of brain neurons and cells scientists focus on. The cortical network in the human brain, being a very complex network, comprises this type of multiscale structure [31,32].
Comparing the basic community detection approach, multi-slice/layer community detection can be used as an evolved version for enhancing its role and features. Especially, some roles such as characterizing time-varying connectivity will be one of the measurements in complex network applications. As a methodological limitation, the multi-slice/layer community detection method will open many types of research and possible future directions.
5.1 Challenges of Complex Networks for Managing Neurons
According to [33], manifold techniques provide some improvement such as complexity reductions in complex network problems and applications. Employing manifold techniques not only reduces the computational complexity but also enhances the energy efficiency between the nodes. It is one of the challenges that could be considered in the future design for managing neurons in brain communication.
In brain communication, mapping the logical model of the brain and its neurological structures [34] allows us to design complex networks for improving the missing nodes and links of the brain and its neurological structures. To improve the EE for energizing neurons, the role of complex networks in brain functions should be efficient with the sensors researchers employed in the systems.
In the intelligent computing system, neuromorphic photonics will provide some features like the human brain. They are information collections through the sensations and process which deals with the interactions of neurons in the brain communication. These features continue with the dynamic approach but processing and storing information continue simultaneously. Therefore, neuromorphic photonics will allow us to analyze the functionality of neurons through sensor technology which involves silicon photonics. Employing IoT-based intelligent computing systems enhances EE and brain communication. The interoperability of neurons can be analyzed via observability recovery which depends on the use of a contraction detection algorithm with complex network properties and a deep learning approach such as ANN.
5.2 Measuring Observability of Neurons’ Status and Connectivity
In observability recovery, the contraction detection algorithm plays an important role with complex network properties and supports measuring the observability of neurons’ status and connectivity. Further, neuroscience offers new opportunities for wireless communications based on 6G developments. On the other hand, 6G wireless technology enhances the features of neuroscience which allow researchers to improve brain communication and healthcare technology including the quick response of viral infection tests [35]. In the future, 6G will control the brain for investigating the defects of the neurons, neuro interactions, etc. monitored during brain communication. One of the key ideas of 6G is wireless brain-machine interactions based on Brain-Machine Interface (BMI) which supports many health devices for measuring the observability of neurons’ status and connectivity during brain-type communication (BTC). Here, Neurosciences for Wireless and Wireless for Neurosciences will open many opportunities they are Brain-Controlled Vehicles (BCV), a new type of service involved with Internet-of-Bio-Nano-Things (IoBNT), etc. As shown in Fig. 2, mapping the logical model of the brain and its neurological structures allows researchers to design complex networks for improving the missing nodes and links of the brain and its neurological structures. These concepts may support the BCV, BMI, and BTC with evolving brain communication.
Figure 2: The computational intelligence model
Technology advances are increasingly combined with powerful network analyzing tools developed in the neuroscience and dynamics of complex systems. This recent confluence of neuroscience and network science allows researchers to investigate brain function from a complex systems perspective which includes complex networks [36–38]. In the human brain, observability of neurons’ status and connectivity can be a model of complex networks with an important admixture of nodes that maintain considerably higher numbers of connections than most other nodes. Human brain networks evolve dynamically with many factors such as age and physical conditions. Complex network analysis of brain and neuron networks has shown that high clustering is often due to the presence of functional modules, or network communities of densely interconnected sensors known as neural elements.
In the 6G development, the concept of neuroscience will enhance the communication capacity of spiking neurons in BTC and spiking networks such as intelligent sensor networks with spiking signals and solutions. In the future, 6G will dominate advanced applications like IoBNT and chaos-based communications. A special example is the BCV where brain signals are used to support the operation of vehicles.
As we mentioned (previous sub-subsection B), measuring the observability of neurons’ status and connectivity depends on many factors; they are such as contraction size, stability of neurons (particularly the neural system model of consciousness as the kernel of natural intelligence and the cognitive model of the brain [38]), etc. According to the future applications, the trends can be divided into the following three categories.
i) Computational Intelligence Model: As shown in Fig. 2, observability depends on the contraction size which allows us to use complex networks influenced by the mathematical model of consciousness. To analyze the observability recovery of neurons’ behaviors (energy performance of all memories (AM), conscious status memory (CSM) of the brain, etc.), we can use a contraction detection algorithm with complex network properties. Observability recovery known as the cognitive memory model of the brain considered in (1) can be defined as
Here, AM contains short-term and long-term memories (STM and LTM) and sensory and action buffer memories (SBM and ABM).
A memory system in a parallel configuration (||) with all memories (2) may be used to formulate the mathematical model of the observability of neurons. Based on [38], the structural model of the brain as autonomous intelligence energy (AIE) which encompasses the brain’s AM and the interaction of neurons depended on a set of the parallel interaction energy between the natural intelligent system (NIS) and the brain’s memory system. Again, AIE can be analyzed with (3) and contraction detection algorithms.
ii) The Observability of Neurons: It can be considered in brain communication for developing an analysis of brain medical issues with the following considerations.
Nanophotonic accelerator designs: Particularly, detailed power or performance modeling of brain communication and connectivity of link quality are required in nanophotonic accelerator designs. Considering heterogeneous computing models based on complex networks, revisiting brain communication and computer architecture for nanophotonic ANN accelerations have to be considered.
Terahertz Communication: Photonic technology and lasers will allow us to build terahertz communication systems [39] and sensors. Still, scanning systems operating with X-rays have many disadvantages. Also, X-rays can cause mutations in our DNA when we analyze medical issues including brain cancer. This ionizing radiation increases the risk of cancer later in life, especially for those exposed to them regularly.
The Complexity of IoT: In the future cyber-physical system (CPS), the scalability of IoT will be increasing with wireless sensors and complex cyber-physical networks (CCN) connecting the social network and health monitoring systems involved with IoT and CPS. A series of models were presented by scholars that allow the analysis and quantification of IoT systems as complex networks [40]. Complexes of nodes and edges increase the computation power and time because matrices of multi-dimensional use a high rank for each calculation. The manifold theory supports reducing the rank of the matrices without losing any performance [41–43]. Specifically, ML such as deep learning applied to graphs usually considers these as manifolds; for this reason, we can consider deep lean learning as a manifold learning challenge.
Security Issues of IoT Communication: As for the security in the complex networks problem, the adversary can exploit the centralization point of SDN-based 5G controllers and attempt to flood the network with massive traffic to overwhelm the network resources. Distributed Denial of Service (DDoS) is an example of such an attack [44,45]. Thus, this attack can be detected by matching the arriving traffic with the predicted one. If the arriving traffic rate is higher than the predicted one, this could be a sign of attack. Consequently, this traffic can be analyzed and classified using the trained eXtreme Gradient Boosting (XGBoost) model to detect and mitigate the attack. Hence, malicious traffic can be detected at an early stage.
Critical Issues of Using Machine Learning (ML) and Deep Learning (DL) models for network security: ML and DL algorithms play an important role in detecting different attack types in various domains such as IoT. In attack detection solutions based on ML and DL models, researchers usually use some publicly available datasets to train their ML and DL models. However, minority class problems could result in misclassification of the traffic which leads to crucial consequences for the network data and recourses. This problem occurs with an imbalanced dataset where some classes have a very small number of instances which is insufficient for the model to be well-trained. Therefore, there is a need to consider such a problem in any future development of datasets. Moreover, sampling methods could be used to overcome the minority class problem, however, it is not an optimal solution for some domains.
With the above considerations, the observability of neurons and their behaviors can be analyzed with energy which supports the interactions of neuron transmissions and receptions. In brain communication, measuring and analyzing the energy variations with the contraction detection algorithm of complex networks will allow researchers to study the energy required for neurons when they are communicating with each other.
iii) Little Brain within the Heart: The heart has an individual manager called a little brain controlled by the big boss which is the brain. This little heart controls the intracardiac nervous system (INS) where neurons play a crucial role in maintaining the heart’s health which allows us to prevent heart disease with early warnings. Employing 3-D mapping and 3-D space of data enhances the controllability of INS which supports the analysis of the heart’s health. Here, complex networks with manifold techniques reduce computational complexity and increase the monitoring speed and controllability of INS. This little brain has many functions related to the heart, but it gets regular communication and induction from the main brain. The little brain can be analyzed using complex networks because INS should be investigated for understanding the details of links and their functional behaviors.
6 Recent Advances and Related Works
Neurocomputer Science: According to [46], observational equivalence in system estimation deals with the observability of complex systems/networks. Measurement of contractions in complex networks depends on the number of nodes which influences the loss of observability. Contraction size also supports the loss of observability as well as observability recovery. To analyze the distribution of the contraction, the authors used the polynomial order contraction detection algorithm. Contraction size related to network clustering coefficient and degree heterogeneity supports reducing the number of measurements. Observational recovery is more restrictive when the system has a few failed sensors or nodes in the system. Limiting the recovery of measurement failure is one of the implications for estimating complex networks and systems. As shown in Fig. 3, neuroscience offers new opportunities for enhancing wireless communications based on 6G. Future e-healthcare applications over wireless networks will depend on the roles of neuroscience. For instance, complex networks with billions of neurons will be supporting to analyze serious medical problems such as brain disorders. Neurons hold billions of years of previous data which may be used for early warning of medical issues.
Figure 3: Wireless for neuroscience and roles of neuroscience for wireless
BRAIN-IoT: Paper [47] showed the details of BRAIN-IoT which includes the model-based framework for dependable sensing and actuation in intelligent decentralized IoT systems. Using BRAIN-IoT, challenges (complex software ecosystem, reacting automatically, runtime to environmental changes, etc.) without human intervention will be improving future autonomous and complex tasks. Furthermore, BRAIN-IoT supports many applications as a general-purpose solution and provides security and privacy protection through the set of components.
Security: As considered in [48], hardware-based encryption and authentication schemes via generalized synchronization of complex networks allow researchers to improve the security of ASIC or FPGA hardware implementation. Further, the authors show that the bit-stream derived from the scheme’s outputs can be used as an encryption or authentication key. Also, they show that regardless of their initial state, implemented hardware receivers synchronize within a short time. They validated the bit-streams generated by the driver using the natural intelligent system test (NIST) suited for randomness and found no deviations.
Neurodynamics: Neuroscience underpins virtually all aspects of neurons’ scientific activities in the brain and focuses on the neuronal signaling and spiking reactions created from the dynamics of neuron communication. Here, the authors sketched the conceptual framework for proposing and analyzing brain networks through communication dynamics. They considered the neurons’ topological attributes of structural networks and functional connectivity involved with interactions between network topology and dynamic communication models [49].
Spreading Efficiency: According to [50], a complex network is an interesting theory that provides an efficient approach to solving complicated problems in which information spreading and details of the spreading efficiency are considered. Although many spreading strategies are available for studying the information spreading within the neuron and brain network, spreading efficiency varies with the different types of networks. Here, the authors proposed solving the cases related to low spreading efficiencies in complicated complex networks. In their proposed approach, they combined two strategies which are small-degree vertices for the specified part and large-degree vertices for the essential part. Further, they claimed that their proposed method was efficient for improving the spreading efficiency.
Roles of IoT: Paper [51] provided the roles of IoT in different products, applications, services, etc., and some backgrounds. The IoT plays an important role in exchanging information according to the users’ requirements and emerging technology. Further, IoT can be easily integrated with many physical systems for measuring natural activities and environmental conditions without human intervention. Here, IoT sensors control the current network infrastructure and other existing systems.
Multiple Complex Networks: According to [52], interdependent networks from a societal perspective are dominating the day-to-day challenges in our life. Here, multiple complex network support enhances the network performance which provides efficient facilities to smart cities included with the many networks such as transport, power, etc. To improve the smart facilities of the network, Multi-Context Influence Tracking on Social Networks (MITS) is introduced. Using the MITS model, social network problems such as coverage, and the ratio of affection metrics was improved.
AI in Complex Networks: Complex event monitoring of sensor data influences the observation of the stress levels of patients through the ANN. In brain communication, recognizing the stress level is one of the key factors for improving the quality of life. Here, ANN was considered for classifying the stress levels and their results through the IoT system which influences the complex event processing capabilities [53].
According to [54], the authors proposed the theoretical model influences the brain’s neurological network (BNN) using big data and emerging complex networks. Although the concept of an IoT network motivates and promotes the facilities of smart cities, the ANN boasts similitude to BNN. Further, ANN plays an important role in the brain network, for instance neuroplasticity which encourages unique complex interactions in a self-learning fashion. In the theoretical model, the BNN with multidimensional interactivity depends on the nature of order and complexity theory.
As mentioned in [55], new features and users’ requirements are investigated for improving the contemporary challenges of 6G. Here, AI plays an important role in enhancing the QoS of 5G applications and 6G architecture development.
According to [56], hybrid intelligence and neural networks support designing AI based on neural networks. Here, hybrid AI is focused on brain-inspired computation systems and applications in the IoT.
Advances of 5G+ and 6G in Complex Networks: Unmanned aerial vehicles (UAVs) are the new wireless components for enhancing future wireless applications such as wireless broadcasting, high-rate wireless services, wireless energy harvesting, etc. In the 5G-Enabled Tactile Internet development, haptics equipment at the edges, along with an unprecedented communications network supports wireless environments and 5G applications [57,58].
According to [59–61], wireless communication systems with AI provide accurate solutions to many challenges that face complicated environments. Despite repeated assurances that the wireless applications are better with 5G+ and 6G, many researchers focus on AI-empowered wireless networks, the wireless communications network for collaborative and AI applications, and edge AI.
Complex Networks with Brain Functions: Complex network analysis has emerged for characterizing brain functions and connectivity relationships. It is an important tool for measuring functional segregation and integration when functional activations are investigated on a local anatomical circuit. Further, the paper [62] focused on quantifying the centrality of individual brain regions or pathways, characterizing patterns of local anatomical circuitry, and testing the resilience of networks to insult.
According to [63], functional systems can be limited based on the quantitative measurements of the brain, but thoughts of the brain cannot be limited and controlled through the functional system. Despite countless thoughts in the brain, the galaxy has countless stars where distances between the stars are varied. Here, features of complex networks allow researchers to analyze the functions of human neuroimaging. In the functional analysis, basic principles of graph theory are introduced to explore the complex brain networks in diverse experimental modalities focused on magnetoencephalography and electroencephalography in humans.
In the paper [64], the authors proposed new measurements of communicability in complex networks in which appropriate shortest paths reach the network destination dynamically. Also, it talks about assortative communicability and the disassortative communicability of complex networks for analyzing the communicability of global systems. In [65], the definition of a new measure is introduced with the weighted communicability measurement applied to complex brain networks.
Advances of SDN, ML, IoT, and Photonics Technology: According to the photonic modulator neuron [66], the silicon photonic process allows the studying of the photonic neural network and understanding of the photonic platforms which create the set of behaviors for a device to act as a neuron participating in a network. Neuromorphic photonics and neurons are growing technology for developing medical devices and engineering with machine intelligence.
Regarding IoT communication, the SDN-based 5G is considered to cope with the massive growth of the number of connected devices as well as the evolving technologies such as IoT-based sensors, Internet of Everything (IoE), CPS, cloud, big data, and act. which results in a drastic increase in traffic load transmitted over the network [67]. The Virtual Network Function (VNF) and SDN are the fundamental technology that 5G relies on to build a flexible architecture that meets the demands of high-speed transmission and low latency services [68]. However, scalability and security are still the main challenges in SDN-based 5G and IoT based 5G+ and beyond. The scalability of the 5G network that meets the variable rate of user equipment attached requests should be performed in a proactive manner instead of a reactive way. Similarly, the security issues that result from the centralization of SDN-based 5G controllers should be addressed proactively as well.
The above-mentioned issues depend on the traffic load variation in complex network problems. To address these problems in SDN-based 5G, there is a need for the anticipation of the traffic load. Once the traffic load is forecasted, the network can be proactively scaled in/out to meet the number of attached requests of the users’ entities. Likewise, malicious traffic can be detected when matching the arrival traffic to the anticipated one. Therefore, a powerful and accurate learning algorithm is needed to forecast the traffic load in SDN-based 5G networks. One of the most powerful and accurate ML algorithms is the eXtreme Gradient Boosting (XGBoost) [69].
The Access and Mobility Management Function (AMF) is the gateway entry component of the 5G network architecture, devised by 3GPP, that manages user equipment registration, authentication, identification, and mobility. AMF can be a bottleneck of the network when the traffic load increases [70]. Therefore, the XGBoost model can be well-trained on a dataset that is generated from the 5G network. Then, this model can be employed on the controllers to forecast the future traffic load. Afterward, the network proactively scales in/out based on the predicted traffic load Thus, the number of AMF can be increased by the VNF when the traffic is forecasted to be increased and vice versa. Therefore, the scalability here is achieved in a proactive way instead of the reactive manner employed in 5G which enhances the network throughput and reduces the drop ratio of attaching requests [71–75]. The IoT ecosystem will have many IoT challenges such as scalability as shown in Fig. 4.
Figure 4: Challenges of IoT with photonic technology
In the future, without photonic sensor technology, the massive deployment of IoT with complex networks would not be possible.
According to the [76–79], routing in complex networks simplifies the efficiency of communication, and connections between the nodes (sensors based on IoT and photonic technology) support measuring the performance of neuron and brain communication. Regarding the future emerging technology derived from the existing work related to this survey, Table 5 shows the summary of all techniques used in this paper.
We studied the role of complex networks in analyzing the scalability of IoT based on 5G communication and the observability of recovery of neurons during brain communication. The scalability of IoT will be possible with 5G or 6G communication based on photonic sensor technology. Although we introduced the 5PSC for improving the external links of brain communication, we have not identified the exact view of internal links, and the design of functional architecture influenced neuron communications and interactions yet.
Whatever the future regarding the viral infection of the human brain and the convergence of new technologies, complex networks will certainly continue for observing the structure and function of the human brain with new analyses.
The artificial neural network structure supports the investigation of the insights of many layers considered in complex networks. Also, ANN enhances the analysis of brain communication using QML which allows us to set the new features of the brain, neurons, and 6G Communication Networks. Here, complex networks based on QML, ML algorithms such as XGBoost, and manifold techniques enhance the users’ requirements of all investigations through the ambitious deep, wide, and complex ANN.
Regarding the IoT and brain communication, the complex networks and the traffic load rate forecasting using the XGBoost algorithm can enhance the performance of the SDN-based 5G networks. The network can proactively scale in/out according to the predicted traffic load which will enhance the performance and remove the delay of the reactive scaling method. Moreover, many security issues can be addressed in SDN-based communication (IoT, IoBNT, etc.) using the XGBoost algorithm by matching the arrival traffic with predicting one and by performing traffic classification for suspected traffic. Thus, employing the XGBoost algorithm enhances the SDN-based 5G network and provides many features such as scalable and flexible resource management and utilization and a secure environment.
The benefits of the complex networks can be applied in future research directions involved with 6G, photonic, IoT, brain, etc. Complex networks will solve many issues dynamically, proactively, intelligently, and instantly with the platform of IoE. It will not only improve communication technologies but also enhance the observability of neuron communication within the brain [80–82].
Acknowledgement: We would like to thank Prof. Santo Fortunato and all members involved in the collaborative project for their valuable advice and continuous support. We would also like to acknowledge the advice and support from the USA-based research group (Computing and Engineering, Indiana University) and the KSA-based research group (Department of Computer Science, King Abdulaziz University).
Funding Statement: This paper was funded by the Deanship of Scientific Research (DSR), King Abdulaziz University, Jeddah, under Grant No. RG-1439-311-10. The authors, therefore, acknowledge with thanks DSR’s technical and financial support.
Author Contributions: The authors confirm contribution to the paper as follows: study conception and design: Vijey Thayananthan, Muhammad Shuaib Qureshi, Hassan A. Alamri, Aiiad Albeshri; data collection: Muhammad Bilal Qureshi, Aiiad Albeshri, Vijey Thayananthan, Hassan A. Alamri; analysis and interpretation of results: Hassan A. Alamri, Vijey Thayananthan, Muhammad Shuaib Qureshi, Muhammad Bilal Qureshi, Aiiad Albeshri; draft manuscript preparation: Vijey Thayananthan, Hassan A. Alamri, Aiiad Albeshri, Muhammad Shuaib Qureshi, Muhammad Bilal Qureshi. All authors reviewed the results and approved the final version of the manuscript.
Availability of Data and Materials: Not applicable.
Conflicts of Interest: The authors declare that they have no conflicts of interest to report regarding the present study.
References
1. Z. Wei, Y. Pan, Q. Shu and S. Meng, “Dynamic development analysis of complex network research: A bibliometric analysis,” Complexity, vol. 2022, 1512939, pp. 16, 2022. [Google Scholar]
2. B. Cristian and M. Pătrașcu, “Engineering emergence: A survey on control in the world of complex networks,” Automation, vol. 3, no. 1, pp. 176–196, 2022. [Google Scholar]
3. D. Rydning, J. Reinsel and J. Gantz, “The digitization of the world from edge to core,” Framingham: International Data Corporation, vol. 16, pp. 1–28, 2018. [Google Scholar]
4. M. Alsharif, A. Kelechi, M. Albreem, S. Chaudhry, M. Zia et al., “Sixth generation (6G) wireless networks: Vision, research activities, challenges, and potential solutions,” Symmetry, vol. 12, no. 4, pp. 676, 2020. [Google Scholar]
5. C. Federico, A. Munari, G. Liva, F. Lazaro, C. Stefanovic et al., “From 5G to 6G: Has the time for modern random access come?” arXiv preprint, arXiv:1903.03063, 2019. [Google Scholar]
6. A. Yasser and E. Hossain, “The D-OMA method for massive multiple access in 6G: Performance, security, and challenges,” IEEE Vehicular Technology Magazine, vol. 14, no. 3, pp. 92–99, 2019. [Google Scholar]
7. Z. E. Ankarali, B. Peköz and H. Arslan, “Flexible radio access beyond 5G: A future projection on the waveform, numerology, and frame design principles,” IEEE Access, vol. 5, pp. 18295–18309, 2017. [Google Scholar]
8. G. Zhiqun, J. Zhang, Y. Ji, L. Bai and X. Sun, “Network topology reconfiguration for FSO-based fronthaul/backhaul in 5G+ wireless networks,” IEEE Access, vol. 6, pp. 69426–69437, 2018. [Google Scholar]
9. N. M. Huda, H. Alves, O. A. López, M. Shehab, P. Diana et al., “Six key enablers for machine type communication in 6G,” arXiv preprint, arXiv.1903.05406, 2019. [Google Scholar]
10. Z. Jun, “A survey of intelligent reflecting surfaces (IRSsTowards 6G wireless communication networks with massive MIMO 2.0,” arXiv preprint, arXiv:1907.04789, 2019. [Google Scholar]
11. X. Huang, J. A. Zhang, R. P. Liu, Y. J. Guo and L. Hanzo, “Airplane-aided integrated networking for 6G wireless: Will it work?” IEEE Vehicular Technology Magazine, vol. 14, no. 3, pp. 84–91, 2019. [Google Scholar]
12. Y. Ping, Y. Xiao, M. Xiao and S. Li, “6G wireless communications: Vision and potential techniques,” IEEE Network, vol. 33, no. 4, pp. 70–75, 2019. [Google Scholar]
13. T. S. Rappaport, Y. Xing, O. Kanhere, S. Ju, A. Madanayake et al., “Wireless communications and applications above 100 GHz: Opportunities and challenges for 6G and beyond,” IEEE Access, vol. 7, pp. 78729–78757, 2019. [Google Scholar]
14. K. Marcos, M. Matinmikko-Blue and M. Latva-Aho, “6Genesis flagship program: Building the bridges towards 6G-enabled wireless smart society and ecosystem,” in Proc. of 2018 IEEE 10th Latin-American Conf. on Communications (LATINCOM), Guadalajara, Mexico, pp. 1–9, 2018. [Google Scholar]
15. T. Aditya, A. Albeshri, V. Thayananthan, W. Alhalabi and S. Fortunato, “Fast consensus clustering in complex networks,” Physical Review, vol. 99, no. 4, pp. 042301, 2019. [Google Scholar]
16. V. Donchenko, A. Trifonova, A. Zemlyanov and R. Ryambov, “Spectral and energy characteristics of resonatorless lasing in solutions of Rhodamine 6G with nanoparticles of various nature with femtosecond pumping,” Journal of Physics, vol. 1499, no. 1, pp. 012020, 2020. [Google Scholar]
17. V. Laurent and L. Pavesi, “Group IV materials,” in Handbook of Silicon Photonics, 1st ed., Boca Raton, Florida, USA: Taylor & Francis, pp. 1–54, 2016. [Google Scholar]
18. J. Goessling, A. González, V. Raj, M. P. Ashworth, S. R. Manning et al., “Biosilica slab photonic crystals as an alternative to cleanroom nanofabrication?” Faraday Discussions, vol. 223, pp. 261–277, 2020. [Google Scholar] [PubMed]
19. S. Nawaz, S. Sharma, S. Wyne, M. Patwary and M. Asaduzzaman, “Quantum machine learning for 6G communication networks: State-of-the-art and vision for the future,” IEEE Access, vol. 7, pp. 46317–46350, 2019. [Google Scholar]
20. G. Marco, M. Polese, M. Mezzavilla, S. Rangan and M. Zorzi, “Toward 6G networks: Use cases and technologies,” IEEE Communications Magazine, vol. 58, no. 3, pp. 55–61, 2020. [Google Scholar]
21. S. Walid, M. Bennis and M. Chen, “A vision of 6G wireless systems: Applications, trends, technologies, and open research problems,” IEEE Network, vol. 34, no. 3, pp. 134–142, 2019. [Google Scholar]
22. Seminars Only, “6G mobile technology,” 2019. [Online]. Available: http://www.123seminarsonly.com/CS/6G-Mobile-Technology.html [Google Scholar]
23. D. Klaus and H. Berndt, “6G vision and requirements: Is there any need for beyond 5G?” IEEE Vehicular Technology Magazine, vol. 13, no. 3, pp. 72–80, 2018. [Google Scholar]
24. F. Tariq, M. Khandaker, K. Wong, M. Imran, M. Bennis et al., “A speculative study on 6G,” IEEE Wireless Communications, vol. 27, no. 4, pp. 118–125, 2020. [Google Scholar]
25. D. C. Nguyen, M. Ding, P. N. Pathirana, A. Seneviratne, J. Li et al., “6G internet of things: A comprehensive survey,” IEEE Internet of Things, vol. 9, no. 1, pp. 359–383, 2021. [Google Scholar]
26. S. Kapil and K. S. Vivek, “Modern architecture for photonic networks-on-chip,” The Journal of Supercomputing, vol. 76, no. 12, pp. 9901–9921, 2020. [Google Scholar]
27. J. Moughames, X. Porte, M. Thiel, G. Ulliac, L. Larger et al., “Three-dimensional waveguide interconnects for scalable integration of photonic neural networks,” Optica, vol. 7, no. 6, pp. 640–646, 2020. [Google Scholar]
28. R. Fard and S. Hosseini, “Machine learning algorithms for prediction of energy consumption and IoT modeling in complex networks,” Microprocessors and Microsystems, vol. 89, pp. 104423, 2022. [Google Scholar]
29. A. Tripathy, S. Chinara and M. Sarkar, “An application of wireless brain–computer interface for drowsiness detection,” Biocybernetics and Biomedical Engineering, vol. 36, no. 1, pp. 276–284, 2016. [Google Scholar]
30. S. Jafri, T. Hamid, R. Mahmood, M. Alam, T. Rafi et al., “Wireless brain computer interface for smart home and medical system,” Wireless Personal Communications, vol. 106, pp. 2163–2177, 2019. [Google Scholar]
31. R. F. Betzel and D. S. Bassett, “Multi-scale brain networks,” Neuroimage, vol. 160, pp. 73–83, 2017. [Google Scholar] [PubMed]
32. V. A. Maksimenko, A. E. Hramov, V. V. Grubov, V. O. Nedaivozov, V. V. Makarov et al., “Nonlinear effect of biological feedback on brain attentional state,” Nonlinear Dynamics, vol. 95, no. 3, pp. 1923–1939, 2019. [Google Scholar]
33. C. Guangfu, C. Xu, J. Wang, J. Feng and J. Feng, “Robust non-negative matrix factorization for link prediction in complex networks using manifold regularization and sparse learning,” Physica A: Statistical Mechanics and its Applications, vol. 539, 122882, 2020. [Google Scholar]
34. Y. Wang, S. Kwong, H. Leung, J. Lu, M. H. Smith et al., “Brain-inspired systems: A transdisciplinary exploration on cognitive cybernetics, humanity, and systems science toward autonomous artificial intelligence,” IEEE Systems, Man, and Cybernetics Magazine, vol. 6, no. 1, pp. 6–13, 2020. [Google Scholar]
35. R. C. Moioli, P. H. J. Nardelli, M. T. Barros, W. Saad, A. Hekmatmanesh et al., “Neurosciences and 6G: Lessons from and needs of communicative brains,” arXiv preprint, arXiv:2004.01834, 2020. [Google Scholar]
36. D. S. Bassett and O. Sporns. “Network neuroscience,” Nature Neuroscience, vol. 20, no. 3, pp. 353–364, 2017. [Google Scholar] [PubMed]
37. B. Hou, Y. Yao and D. Liao, “Identifying all-around nodes for spreading dynamics in complex networks,” Physica, vol. 391, pp. 4012–4017, 2012. [Google Scholar]
38. M. Rubinov and O. Sporns, “Complex network measures of brain connectivity: Uses and interpretations,” Neuro Image, vol. 52, pp. 1059–1069, 2010. [Google Scholar] [PubMed]
39. S. Dhillon, M. Vitiello, E. Linfield, A. Davies, M. Hoffmann et al., “The 2017 terahertz science and technology roadmap,” Journal of Physics, vol. 50, pp. 043001, 2017. [Google Scholar]
40. J. Villalba, “The lean brain theory,” in Complex Networked Lean Strategic Organizational Design. Boca Raton, FL, USA: CRC Press, 2017. [Google Scholar]
41. Z. Wu, G. Menichetti, C. Rahmede and G. Bianconi, “Emergent complex network geometry,” Scientific. Reports, vol. 5, 10073, 2015. [Google Scholar]
42. G. Bianconi, C. Rahmede and Z. Wu, “Complex quantum network geometries: Evolution and phase transitions,” Physical Review E, vol. 92, 022815, 2015. [Google Scholar]
43. G. Bianconi and C. Rahmede, “Complex quantum network manifolds in dimension d > 2 are scale-free,” Scientific Reports, vol. 5, 13979, 2015. [Google Scholar]
44. M. K. Forland, K. Kralevska, M. Garau and D. Gligoroski, “Preventing DDoS with SDN in 5G,” in Proc. of 2019 IEEE Globecom Workshops, Waikoloa, HI, USA, pp. 1–7, 2019. [Google Scholar]
45. K. Cabaj, M. Gregorczyk, W. Mazurczyk, P. Nowakowski and P. Zorawski, “SDN-based mitigation of scanning attacks for the 5G internet of radio light system,” in Proc. of Int. Conf. on Availability, Reliability and Security, ACM, vol. 49, pp. 1–10, 2018. [Google Scholar]
46. M. Doostmohammadian, H. R. Rabiee, H. Zarrabi and U. Khan, “Observational equivalence in system estimation: Contractions in complex networks,” IEEE Transactions on Network Science and Engineering, vol. 5, no. 3, pp. 212–224, 2017. [Google Scholar]
47. D. Conzon, M. R. A. Rashid, X. Tao, A. Soriano, R. Nicholson et al., “BRAIN-IoT: Model-based framework for dependable sensing and actuation in intelligent decentralized IoT systems,” in Proc. of 2019 4th Int. Conf. on Computing, Communications and Security (ICCCS), Rome, Italy, pp. 1–8, 2019. [Google Scholar]
48. L. Keuninckx and G. Sande, “Hardware-based encryption via generalized synchronization of complex networks,” in IoT Security: Advances in Authentication, 1st ed., Hoboken, New Jersey, USA: Wiley Telecom, pp. 205–224, 2020. [Google Scholar]
49. A. Andrea, B. Misic and O. Sporns, “Communication dynamics in complex brain networks,” Nature Reviews Neuroscience, vol. 19, no. 1, pp. 17–33, 2017. [Google Scholar]
50. W. Shuangyan, W. Cheng and G. Mei, “Efficient method for improving the spreading efficiency in small-world networks and assortative scale-free networks,” IEEE Access, vol. 7, pp. 46122–46134, 2019. [Google Scholar]
51. K. Jozsef, T. Ujbanyi, G. Sziladi and A. Kovari, “Electroencephalogram-based brain-computer interface for internet of robotic things,” in Cognitive Infocommunications, Theory and Applications, 1st ed., Cham, Switzerland: Springer, pp. 253–275, 2019. [Google Scholar]
52. R. Baral, S. Iyengar and A. Madni, “Interdependent networks from societal perspective: MITS (multi-context influence tracking on social network),” in Sustainable Interdependent Networks, 2nd eds., Cham, Switzerland: Springer, pp. 1–22, 2019. [Google Scholar]
53. D. Marković, D., Vujičić, D. Stojić, Ž. Jovanović, U. Pešović et al., “Monitoring system based on IoT sensor data with complex event processing and artificial neural networks for patients stress detection,” in Proc. of 2019 18th Int. Symp. INFOTEH-JAHORINA (INFOTEH), East Sarajevo, Bosnia and Herzegovina, pp. 1–6, 2019. [Google Scholar]
54. A. Zaheer, “Achieving neuroplasticity in artificial neural networks through smart cities,” Smart Cities, vol. 2, no. 2, pp. 118–134, 2019. [Google Scholar]
55. M. Z. Chowdhury, M. Shahjalal, S. Ahmed and Y. M. Jang, “6G wireless communication systems: Applications, requirements, technologies, challenges, and research directions,” IEEE Open Journal of the Communications Society, vol. 1, pp. 957–975, 2020. [Google Scholar]
56. Z. H. Guan, B. Hu and X. Shen, “Hybrid intelligent networks,” in Introduction to Hybrid Intelligent Networks: Modeling, Communication, and Control, 1st ed., Cham, Switzerland: Springer, pp. 1–26, 2019. [Google Scholar]
57. L. Bin, Z. Fei and Y. Zhang, “UAV communications for 5G and beyond: Recent advances and future trends,” IEEE Internet of Things, vol. 6, no. 2, pp. 2241–2263, 2018. [Google Scholar]
58. P. R. Newswire, “5G and the haptic internet: Emerging technologies, solutions and market opportunities,” 2019. [Online]. Available: https://www.prnewswire.com/news-releases/5g-and-the-haptic-internet-emerging-technologies-solutions-and-market-opportunities-300184874.html [Google Scholar]
59. K. B. Letaief, W. Chen, Y. Shi, J. Zhang and Y. J. A. Zhang, “The roadmap to 6G: AI empowered wireless networks,” IEEE Communications Magazine, vol. 57, no. 8, pp. 84–90, 2019. [Google Scholar]
60. R. A. Stoica and G. Abreu, “6G: The wireless communications network for collaborative and AI applications,” arXiv preprint, arXiv:1904.03413, 2019. [Google Scholar]
61. L. Lovén, T. Leppänen, E. Peltonen, J. Partala, E. Harjula et al., “EdgeAI: A vision for distributed, edge-native artificial intelligence in future 6G networks,” in Proc. of the 1st 6G Wireless Summit, Levi, Finland, pp. 1–2, 2019. [Google Scholar]
62. R. Mikail and O. Sporns, “Complex network measures of brain connectivity, uses and interpretations,” Neuroimage, vol. 52, no. 3, pp. 1059–1069, 2010. [Google Scholar]
63. B. Ed and O. Sporns, “Complex brain networks: Graph theoretical analysis of structural and functional systems,” Nature Reviews Neuroscience, vol. 10, no. 3, pp. 186–198, 2009. [Google Scholar]
64. E. Ernesto and N. Hatano, “Communicability in complex networks,” Physical Review, vol. 77, no. 3, pp. 036111, 2008. [Google Scholar]
65. J. Crofts and D. J. Higham, “A weighted communicability measure applied to complex brain networks,” Journal of the Royal Society Interface, vol. 6, no. 33, pp. 411–414, 2009. [Google Scholar] [PubMed]
66. A. Tait, T. De Lima, M. Nahmias, H. Tait, H. Peng et al., “Silicon photonic modulator neuron,” Physical Review, vol. 11, no. 6, pp. 064043, 2019. [Google Scholar]
67. L. V. Le, D. Sinh, B. Lin and L. P. Tung, “Applying big data, machine learning, and SDN/NFV to 5G traffic clustering, forecasting, and management,” in Proc. of 2018 4th IEEE Conf. on Network Softwarization and Workshops (NetSoft), Montreal, QC, Canada, pp. 168–176, 2018. [Google Scholar]
68. I. Alawe, A. Ksentini, Y. Hadjadj and P. Bertin, “Improving traffic forecasting for 5G core network scalability: A machine learning approach,” IEEE Network, vol. 32, no. 6, pp. 42–49, 2018. [Google Scholar]
69. T. Chen and C. Guestrin, “Xgboost: A scalable tree boosting system,” in Proc. 22nd ACM Sigkdd Int. Conf. on Knowledge Discovery and Data Mining, San Francisco, California, USA, 13–17 August, pp. 785–794, 2016. [Google Scholar]
70. I. Alawe, Y. Hadjadj, A. Ksentini, P. Bertin and D. Darche, “On the scalability of 5G core network: The AMF case,” in Proc. of 2018 15th IEEE Annual Consumer Communications & Networking Conf. (CCNC), Las Vegas, NV, USA, pp. 1–6, 2018. [Google Scholar]
71. Europe’s Age of Light—Strategic Roadmap March 2019, Photonics21.org, 2019. [Online]. Available: https://www.photonics21.org/download/ppp-services/photonics-downloads/Europes-age-of-light-Photonics-Roadmap-C1.pdf [Google Scholar]
72. A. Viol, F. Palhano, H. Onias, D. Araujo and G. M. Viswanathan, “Shannon entropy of brain functional complex networks under the influence of the psychedelic ayahuasca,” Scientific Reports, vol. 7, 7388, 2017. [Google Scholar]
73. M. Stella and M. Domenico, “Distance entropy cartography characterizes centrality in complex networks,” Entropy, vol. 20, no. 4, pp. 268, 2018. [Google Scholar] [PubMed]
74. D. Papo, “Commentary: The entropic brain: A theory of conscious states informed by neuroimaging research with psychedelic drugs,” Frontiers in Human Neuroscience, vol. 10, pp. 423, 2016. [Google Scholar] [PubMed]
75. S. Bansal and D. Kumar, “IoT ecosystem: A survey on devices, gateways, operating systems, middleware and communication,” International Journal of Wireless Information Networks, vol. 27, no. 4, pp. 340–364, 2020. [Google Scholar]
76. A. Viol, F. Palhano-Fontes, H. Onias, D. B. Araujo, P. Hövel et al., “Characterizing complex networks using entropy-degree diagrams: Unveiling changes in functional brain connectivity induced by Ayahuasca,” Entropy, vol. 21, no. 2, pp. 1–28, 2019. [Google Scholar]
77. G. Yan, T. Zhou, B. Hu, Z. Q. Fu and B. H. Wang, “Efficient routing on complex networks,” Physical Review, vol. 73, no. 4, pp. 046108, 2006. [Google Scholar] [PubMed]
78. A. Muscoloni and C. V. Cannistraci, “Navigability evaluation of complex networks by greedy routing efficiency,” National Academy of Sciences, vol. 116, no. 5, pp. 1468–1469, 2019. [Google Scholar]
79. J. Chen, J. Cao and W. Huang, “Traffic-driven explosive synchronization with adaptive local routing in complex networks,” Chaos, Solitons and Fractals, vol. 168, pp. 113–142, 2023. [Google Scholar]
80. T. Pereira, J. Impagliazzo and H. Santos, “Internet of everything,” in Proc. of First EAI Int. Conf., IoECon 2022, Guimarães, Portugal, Springer Nature, vol. 458, 2022. [Google Scholar]
81. CISCO, “The internet of everything,” 2019. [Online]. Available: https://www.cisco.com/c/dam/en_us/about/business-insights/docs/ioe-value-at-stake-public-sector-analysis-faq.pdf [Google Scholar]
82. G. Liu, Y. Huang, N. Li, J. Dong, J. Jin et al., “Vision, requirements and network architecture of 6G mobile network beyond 2030,” China Communications, vol. 17, no. 9, pp. 92–104, 2020. [Google Scholar]
Cite This Article
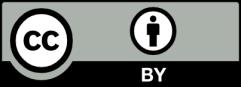