Open Access
ARTICLE
DT-Net: Joint Dual-Input Transformer and CNN for Retinal Vessel Segmentation
1 School of Information Science and Technology, Shijiazhuang Tiedao University, Shijiazhuang, China
2 School of Mathematics and Information Science, Zhangjiakou University, Zhangjiakou, China
* Corresponding Author: Yan Sun. Email:
(This article belongs to the Special Issue: Recent Advances in Ophthalmic Diseases Diagnosis using AI)
Computers, Materials & Continua 2023, 76(3), 3393-3411. https://doi.org/10.32604/cmc.2023.040091
Received 04 March 2023; Accepted 28 June 2023; Issue published 08 October 2023
Abstract
Retinal vessel segmentation in fundus images plays an essential role in the screening, diagnosis, and treatment of many diseases. The acquired fundus images generally have the following problems: uneven illumination, high noise, and complex structure. It makes vessel segmentation very challenging. Previous methods of retinal vascular segmentation mainly use convolutional neural networks on U Network (U-Net) models, and they have many limitations and shortcomings, such as the loss of microvascular details at the end of the vessels. We address the limitations of convolution by introducing the transformer into retinal vessel segmentation. Therefore, we propose a hybrid method for retinal vessel segmentation based on modulated deformable convolution and the transformer, named DT-Net. Firstly, multi-scale image features are extracted by deformable convolution and multi-head self-attention (MHSA). Secondly, image information is recovered, and vessel morphology is refined by the proposed transformer decoder block. Finally, the local prediction results are obtained by the side output layer. The accuracy of the vessel segmentation is improved by the hybrid loss function. Experimental results show that our method obtains good segmentation performance on Specificity (SP), Sensitivity (SE), Accuracy (ACC), Curve (AUC), and F1-score on three publicly available fundus datasets such as DRIVE, STARE, and CHASE_DB1.Keywords
Cite This Article
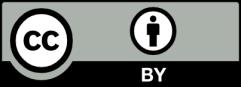
This work is licensed under a Creative Commons Attribution 4.0 International License , which permits unrestricted use, distribution, and reproduction in any medium, provided the original work is properly cited.