Open Access
ARTICLE
Multi-Agent Deep Reinforcement Learning for Efficient Computation Offloading in Mobile Edge Computing
Department of Software Engineering, Software College, Northeastern University, Shenyang, 110819, China
* Corresponding Author: Jie Song. Email:
Computers, Materials & Continua 2023, 76(3), 3585-3603. https://doi.org/10.32604/cmc.2023.040068
Received 03 March 2023; Accepted 18 July 2023; Issue published 08 October 2023
Abstract
Mobile-edge computing (MEC) is a promising technology for the fifth-generation (5G) and sixth-generation (6G) architectures, which provides resourceful computing capabilities for Internet of Things (IoT) devices, such as virtual reality, mobile devices, and smart cities. In general, these IoT applications always bring higher energy consumption than traditional applications, which are usually energy-constrained. To provide persistent energy, many references have studied the offloading problem to save energy consumption. However, the dynamic environment dramatically increases the optimization difficulty of the offloading decision. In this paper, we aim to minimize the energy consumption of the entire MEC system under the latency constraint by fully considering the dynamic environment. Under Markov games, we propose a multi-agent deep reinforcement learning approach based on the bi-level actor-critic learning structure to jointly optimize the offloading decision and resource allocation, which can solve the combinatorial optimization problem using an asymmetric method and compute the Stackelberg equilibrium as a better convergence point than Nash equilibrium in terms of Pareto superiority. Our method can better adapt to a dynamic environment during the data transmission than the single-agent strategy and can effectively tackle the coordination problem in the multi-agent environment. The simulation results show that the proposed method could decrease the total computational overhead by 17.8% compared to the actor-critic-based method and reduce the total computational overhead by 31.3%, 36.5%, and 44.7% compared with random offloading, all local execution, and all offloading execution, respectively.Keywords
Cite This Article
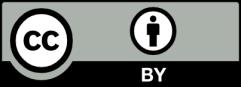
This work is licensed under a Creative Commons Attribution 4.0 International License , which permits unrestricted use, distribution, and reproduction in any medium, provided the original work is properly cited.