Open Access
ARTICLE
A Comprehensive Analysis of Datasets for Automotive Intrusion Detection Systems
1 School of Cybersecurity, Korea University, Seoul, 02841, Korea
2 Memory Division, Samsung Electronics, Hwaseong, 18449, Korea
* Corresponding Author: Dong Hoon Lee. Email:
(This article belongs to the Special Issue: Advances in Information Security Application)
Computers, Materials & Continua 2023, 76(3), 3413-3442. https://doi.org/10.32604/cmc.2023.039583
Received 06 February 2023; Accepted 21 June 2023; Issue published 08 October 2023
Abstract
Recently, automotive intrusion detection systems (IDSs) have emerged as promising defense approaches to counter attacks on in-vehicle networks (IVNs). However, the effectiveness of IDSs relies heavily on the quality of the datasets used for training and evaluation. Despite the availability of several datasets for automotive IDSs, there has been a lack of comprehensive analysis focusing on assessing these datasets. This paper aims to address the need for dataset assessment in the context of automotive IDSs. It proposes qualitative and quantitative metrics that are independent of specific automotive IDSs, to evaluate the quality of datasets. These metrics take into consideration various aspects such as dataset description, collection environment, and attack complexity. This paper evaluates eight commonly used datasets for automotive IDSs using the proposed metrics. The evaluation reveals biases in the datasets, particularly in terms of limited contexts and lack of diversity. Additionally, it highlights that the attacks in the datasets were mostly injected without considering normal behaviors, which poses challenges for training and evaluating machine learning-based IDSs. This paper emphasizes the importance of addressing the identified limitations in existing datasets to improve the performance and adaptability of automotive IDSs. The proposed metrics can serve as valuable guidelines for researchers and practitioners in selecting and constructing high-quality datasets for automotive security applications. Finally, this paper presents the requirements for high-quality datasets, including the need for representativeness, diversity, and balance.Keywords
Cite This Article
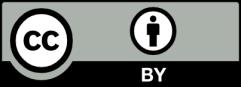