Open Access
ARTICLE
Image Steganalysis Based on Deep Content Features Clustering
1 Henan Provincial Key Laboratory of Cyberspace Situational Awareness, Zhengzhou, 450001, China
2 Zhengzhou Science and Technology Institute, Zhengzhou, 450001, China
3 School of Science, Aalto University, Espoo, 02150, Finland
* Corresponding Author: Ma Zhu. Email:
Computers, Materials & Continua 2023, 76(3), 2921-2936. https://doi.org/10.32604/cmc.2023.039540
Received 03 February 2023; Accepted 12 April 2023; Issue published 08 October 2023
Abstract
The training images with obviously different contents to the detected images will make the steganalysis model perform poorly in deep steganalysis. The existing methods try to reduce this effect by discarding some features related to image contents. Inevitably, this should lose much helpful information and cause low detection accuracy. This paper proposes an image steganalysis method based on deep content features clustering to solve this problem. Firstly, the wavelet transform is used to remove the high-frequency noise of the image, and the deep convolutional neural network is used to extract the content features of the low-frequency information of the image. Then, the extracted features are clustered to obtain the corresponding class labels to achieve sample pre-classification. Finally, the steganalysis network is trained separately using samples in each subclass to achieve more reliable steganalysis. We experimented on publicly available combined datasets of Bossbase1.01, Bows2, and ALASKA#2 with a quality factor of 75. The accuracy of our proposed pre-classification scheme can improve the detection accuracy by 4.84% for Joint Photographic Experts Group UNIversal WAvelet Relative Distortion (J-UNIWARD) at the payload of 0.4 bits per non-zero alternating current discrete cosine transform coefficient (bpnzAC). Furthermore, at the payload of 0.2 bpnzAC, the improvement effect is minimal but also reaches 1.39%. Compared with the previous steganalysis based on deep learning, this method considers the differences between the training contents. It selects the proper detector for the image to be detected. Experimental results show that the pre-classification scheme can effectively obtain image subclasses with certain similarities and better ensure the consistency of training and testing images. The above measures reduce the impact of sample content inconsistency on the steganalysis network and improve the accuracy of steganalysis.Keywords
Cite This Article
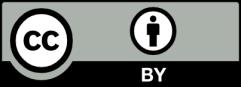