Open Access
ARTICLE
FedTC: A Personalized Federated Learning Method with Two Classifiers
1 School of Computer Science and Technology, Harbin Institute of Technology, Shenzhen, 518055, China
2 Guangdong Provincial Key Laboratory of Novel Security Intelligence Technologies, Shenzhen, 518055, China
3 Research Center for Cyberspace Security, Peng Cheng Laboratory, Shenzhen, 518055, China
* Corresponding Authors: Jiabo Wang. Email: ; Jiajia Zhang. Email:
(This article belongs to the Special Issue: Advances in Information Security Application)
Computers, Materials & Continua 2023, 76(3), 3013-3027. https://doi.org/10.32604/cmc.2023.039452
Received 31 January 2023; Accepted 29 April 2023; Issue published 08 October 2023
Abstract
Centralized training of deep learning models poses privacy risks that hinder their deployment. Federated learning (FL) has emerged as a solution to address these risks, allowing multiple clients to train deep learning models collaboratively without sharing raw data. However, FL is vulnerable to the impact of heterogeneous distributed data, which weakens convergence stability and suboptimal performance of the trained model on local data. This is due to the discarding of the old local model at each round of training, which results in the loss of personalized information in the model critical for maintaining model accuracy and ensuring robustness. In this paper, we propose FedTC, a personalized federated learning method with two classifiers that can retain personalized information in the local model and improve the model’s performance on local data. FedTC divides the model into two parts, namely, the extractor and the classifier, where the classifier is the last layer of the model, and the extractor consists of other layers. The classifier in the local model is always retained to ensure that the personalized information is not lost. After receiving the global model, the local extractor is overwritten by the global model’s extractor, and the classifier of the global model serves as an additional classifier of the local model to guide local training. The FedTC introduces a two-classifier training strategy to coordinate the two classifiers for local model updates. Experimental results on Cifar10 and Cifar100 datasets demonstrate that FedTC performs better on heterogeneous data than current studies, such as FedAvg, FedPer, and local training, achieving a maximum improvement of 27.95% in model classification test accuracy compared to FedAvg.Keywords
Cite This Article
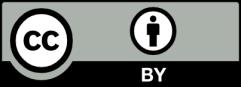
This work is licensed under a Creative Commons Attribution 4.0 International License , which permits unrestricted use, distribution, and reproduction in any medium, provided the original work is properly cited.