Open Access
ARTICLE
Self-Awakened Particle Swarm Optimization BN Structure Learning Algorithm Based on Search Space Constraint
1 School of Information and Communication Engineering, Hainan University, Haikou, 570228, China
2 Playload Research Center for Space Electronic Information System, Academy of Space Electronic Information Technology, Xi’an, 710100, China
3 Research Center, CRSC Communication & Information Group Co., Ltd., Beijing, 100070, China
* Corresponding Author: Yu Zhang. Email:
(This article belongs to the Special Issue: Evolving Network Traffic Identification Technology)
Computers, Materials & Continua 2023, 76(3), 3257-3274. https://doi.org/10.32604/cmc.2023.039430
Received 29 January 2023; Accepted 05 June 2023; Issue published 08 October 2023
Abstract
To obtain the optimal Bayesian network (BN) structure, researchers often use the hybrid learning algorithm that combines the constraint-based (CB) method and the score-and-search (SS) method. This hybrid method has the problem that the search efficiency could be improved due to the ample search space. The search process quickly falls into the local optimal solution, unable to obtain the global optimal. Based on this, the Particle Swarm Optimization (PSO) algorithm based on the search space constraint process is proposed. In the first stage, the method uses dynamic adjustment factors to constrain the structure search space and enrich the diversity of the initial particles. In the second stage, the update mechanism is redefined, so that each step of the update process is consistent with the current structure which forms a one-to-one correspondence. At the same time, the “self-awakened” mechanism is added to prevent precocious particles from being part of the best. After the fitness value of the particle converges prematurely, the activation operation makes the particles jump out of the local optimal values to prevent the algorithm from converging too quickly into the local optimum. Finally, the standard network dataset was compared with other algorithms. The experimental results showed that the algorithm could find the optimal solution at a small number of iterations and a more accurate network structure to verify the algorithm’s effectiveness.Keywords
Cite This Article
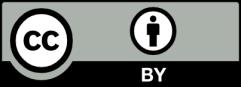