Open Access
ARTICLE
An Improved Jump Spider Optimization for Network Traffic Identification Feature Selection
School of Computer Science, Hubei University of Technology, Wuhan, 430068, China
* Corresponding Author: Yalin Hu. Email:
(This article belongs to the Special Issue: Evolving Network Traffic Identification Technology)
Computers, Materials & Continua 2023, 76(3), 3239-3255. https://doi.org/10.32604/cmc.2023.039227
Received 16 January 2023; Accepted 18 May 2023; Issue published 08 October 2023
Abstract
The massive influx of traffic on the Internet has made the composition of web traffic increasingly complex. Traditional port-based or protocol-based network traffic identification methods are no longer suitable for today’s complex and changing networks. Recently, machine learning has been widely applied to network traffic recognition. Still, high-dimensional features and redundant data in network traffic can lead to slow convergence problems and low identification accuracy of network traffic recognition algorithms. Taking advantage of the faster optimization-seeking capability of the jumping spider optimization algorithm (JSOA), this paper proposes a jumping spider optimization algorithm that incorporates the harris hawk optimization (HHO) and small hole imaging (HHJSOA). We use it in network traffic identification feature selection. First, the method incorporates the HHO escape energy factor and the hard siege strategy to form a new search strategy for HHJSOA. This location update strategy enhances the search range of the optimal solution of HHJSOA. We use small hole imaging to update the inferior individual. Next, the feature selection problem is coded to propose a jumping spiders individual coding scheme. Multiple iterations of the HHJSOA algorithm find the optimal individual used as the selected feature for KNN classification. Finally, we validate the classification accuracy and performance of the HHJSOA algorithm using the UNSW-NB15 dataset and KDD99 dataset. Experimental results show that compared with other algorithms for the UNSW-NB15 dataset, the improvement is at least 0.0705, 0.00147, and 1 on the accuracy, fitness value, and the number of features. In addition, compared with other feature selection methods for the same datasets, the proposed algorithm has faster convergence, better merit-seeking, and robustness. Therefore, HHJSOA can improve the classification accuracy and solve the problem that the network traffic recognition algorithm needs to be faster to converge and easily fall into local optimum due to high-dimensional features.Keywords
Cite This Article
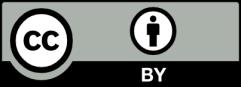
This work is licensed under a Creative Commons Attribution 4.0 International License , which permits unrestricted use, distribution, and reproduction in any medium, provided the original work is properly cited.