Open Access
ARTICLE
A Transmission and Transformation Fault Detection Algorithm Based on Improved YOLOv5
1 School of Information Science and Engineering, Hebei University of Science and Technology, Shijiazhuang, 050000, China
2 Department of Computer Science and Information Systems, Birkbeck Institute for Data Analytics, London,B100AB WC1E 7HX, UK
* Corresponding Author: Jingfang Su. Email:
Computers, Materials & Continua 2023, 76(3), 2997-3011. https://doi.org/10.32604/cmc.2023.038923
Received 04 January 2023; Accepted 29 April 2023; Issue published 08 October 2023
Abstract
On the transmission line, the invasion of foreign objects such as kites, plastic bags, and balloons and the damage to electronic components are common transmission line faults. Detecting these faults is of great significance for the safe operation of power systems. Therefore, a YOLOv5 target detection method based on a deep convolution neural network is proposed. In this paper, Mobilenetv2 is used to replace Cross Stage Partial (CSP)-Darknet53 as the backbone. The structure uses depth-wise separable convolution toreduce the amount of calculation and parameters; improve the detection rate. At the same time, to compensate for the detection accuracy, the Squeeze-and-Excitation Networks (SENet) attention model is fused into the algorithm framework and a new detection scale suitable for small targets is added to improve the significance of the fault target area in the image. Collect pictures of foreign matters such as kites, plastic bags, balloons, and insulator defects of transmission lines, and sort them into a data set. The experimental results on datasets show that the mean Accuracy Precision (mAP) and recall rate of the algorithm can reach 92.1% and 92.4%, respectively. At the same time, by comparison, the detection accuracy of the proposed algorithm is higher than that of other methods.Keywords
Cite This Article
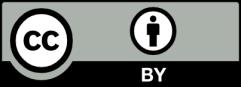
This work is licensed under a Creative Commons Attribution 4.0 International License , which permits unrestricted use, distribution, and reproduction in any medium, provided the original work is properly cited.