Open Access
ARTICLE
AI-Driven FBMC-OQAM Signal Recognition via Transform Channel Convolution Strategy
1 School of Communication and Information Engineering, Chongqing University of Posts and Telecommunications, Chongqing, 400065, China
2 Department of Electronic Systems, Aalborg University, Aalborg, DK 9220, Denmark
* Corresponding Author: Tianqi Zhang. Email:
Computers, Materials & Continua 2023, 76(3), 2817-2834. https://doi.org/10.32604/cmc.2023.037832
Received 18 November 2022; Accepted 13 February 2023; Issue published 08 October 2023
Abstract
With the advent of the Industry 5.0 era, the Internet of Things (IoT) devices face unprecedented proliferation, requiring higher communications rates and lower transmission delays. Considering its high spectrum efficiency, the promising filter bank multicarrier (FBMC) technique using offset quadrature amplitude modulation (OQAM) has been applied to Beyond 5G (B5G) industry IoT networks. However, due to the broadcasting nature of wireless channels, the FBMC-OQAM industry IoT network is inevitably vulnerable to adversary attacks from malicious IoT nodes. The FBMC-OQAM industry cognitive radio network (ICRNet) is proposed to ensure security at the physical layer to tackle the above challenge. As a pivotal step of ICRNet, blind modulation recognition (BMR) can detect and recognize the modulation type of malicious signals. The previous works need to accomplish the BMR task of FBMC-OQAM signals in ICRNet nodes. A novel FBMC BMR algorithm is proposed with the transform channel convolution network (TCCNet) rather than a complicated two-dimensional convolution. Firstly, this is achieved by designing a low-complexity binary constellation diagram (BCD) gridding matrix as the input of TCCNet. Then, a transform channel convolution strategy is developed to convert the image-like BCD matrix into a series-like data format, accelerating the BMR process while keeping discriminative features. Monte Carlo experimental results demonstrate that the proposed TCCNet obtains a performance gain of 8% and 40% over the traditional in-phase/quadrature (I/Q)-based and constellation diagram (CD)-based methods at a signal noise ratio (SNR) of 12 dB, respectively. Moreover, the proposed TCCNet can achieve around 29.682 and 2.356 times faster than existing CD-Alex Network (CD-AlexNet) and I/Q-Convolutional Long Deep Neural Network (I/Q-CLDNN) algorithms, respectively.Keywords
Cite This Article
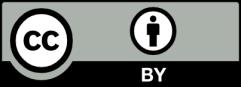
This work is licensed under a Creative Commons Attribution 4.0 International License , which permits unrestricted use, distribution, and reproduction in any medium, provided the original work is properly cited.