Open Access
ARTICLE
CNN Based Features Extraction and Selection Using EPO Optimizer for Cotton Leaf Diseases Classification
1 Department of Computer Science, COMSATS University Islamabad, Wah Campus, Wah Cantt, 47040, Pakistan
2 Department of Computer Science, University of Wah, Wah Cantt, 47040, Pakistan
3 National University of Technology (NUTECH), Islamabad, 44000, Pakistan
4 Department of Applied Data Science, Noroff University College, Kristiansand, Norway
5 Artificial Intelligence Research Center (AIRC), College of Engineering and Information Technology, Ajman University, Ajman, United Arab Emirates
6 Department of Electrical and Computer Engineering, Lebanese American University, Byblos, Lebanon
7 Department of Software, Kongju National University, Cheonan, 31080, Korea
* Corresponding Author: Jungeun Kim. Email:
(This article belongs to the Special Issue: Recent Advances in Deep Learning and Saliency Methods for Agriculture)
Computers, Materials & Continua 2023, 76(3), 2779-2793. https://doi.org/10.32604/cmc.2023.035860
Received 07 September 2022; Accepted 15 January 2023; Issue published 08 October 2023
Abstract
Worldwide cotton is the most profitable cash crop. Each year the production of this crop suffers because of several diseases. At an early stage, computerized methods are used for disease detection that may reduce the loss in the production of cotton. Although several methods are proposed for the detection of cotton diseases, however, still there are limitations because of low-quality images, size, shape, variations in orientation, and complex background. Due to these factors, there is a need for novel methods for features extraction/selection for the accurate cotton disease classification. Therefore in this research, an optimized features fusion-based model is proposed, in which two pre-trained architectures called EfficientNet-b0 and Inception-v3 are utilized to extract features, each model extracts the feature vector of length N 1000. After that, the extracted features are serially concatenated having a feature vector length N 2000. The most prominent features are selected using Emperor Penguin Optimizer (EPO) method. The method is evaluated on two publically available datasets, such as Kaggle cotton disease dataset-I, and Kaggle cotton-leaf-infection-II. The EPO method returns the feature vector of length 1 755, and 1 824 using dataset-I, and dataset-II, respectively. The classification is performed using 5, 7, and 10 folds cross-validation. The Quadratic Discriminant Analysis (QDA) classifier provides an accuracy of 98.9% on 5 fold, 98.96% on 7 fold, and 99.07% on 10 fold using Kaggle cotton disease dataset-I while the Ensemble Subspace K Nearest Neighbor (KNN) provides 99.16% on 5 fold, 98.99% on 7 fold, and 99.27% on 10 fold using Kaggle cotton-leaf-infection dataset-II.Keywords
Cite This Article
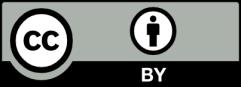
This work is licensed under a Creative Commons Attribution 4.0 International License , which permits unrestricted use, distribution, and reproduction in any medium, provided the original work is properly cited.