Open Access
ARTICLE
Deep Learning Models Based on Weakly Supervised Learning and Clustering Visualization for Disease Diagnosis
1 School of Computer and Information Engineering, Chuzhou University, Chuzhou, 239000, China
2 School of Computer Science and Technology, Changchun University of Science and Technology, Changchun,
130022, China
3 Zhongshan Institute of Changchun University of Science and Technology, Zhongshan, China
4 School of Intelligent Engineering, Henan Institute of Technology, Xinxiang, 453003, China
* Corresponding Author: Zhengang Jiang. Email:
Computers, Materials & Continua 2023, 76(3), 2649-2665. https://doi.org/10.32604/cmc.2023.038891
Received 02 January 2023; Accepted 17 May 2023; Issue published 08 October 2023
Abstract
The coronavirus disease 2019 (COVID-19) has severely disrupted both human life and the health care system. Timely diagnosis and treatment have become increasingly important; however, the distribution and size of lesions vary widely among individuals, making it challenging to accurately diagnose the disease. This study proposed a deep-learning disease diagnosis model based on weakly supervised learning and clustering visualization (W_CVNet) that fused classification with segmentation. First, the data were preprocessed. An optimizable weakly supervised segmentation preprocessing method (O-WSSPM) was used to remove redundant data and solve the category imbalance problem. Second, a deep-learning fusion method was used for feature extraction and classification recognition. A dual asymmetric complementary bilinear feature extraction method (D-CBM) was used to fully extract complementary features, which solved the problem of insufficient feature extraction by a single deep learning network. Third, an unsupervised learning method based on Fuzzy C-Means (FCM) clustering was used to segment and visualize COVID-19 lesions enabling physicians to accurately assess lesion distribution and disease severity. In this study, 5-fold crossvalidation methods were used, and the results showed that the network had an average classification accuracy of 85.8%, outperforming six recent advanced classification models. W_CVNet can effectively help physicians with automated aid in diagnosis to determine if the disease is present and, in the case of COVID-19 patients, to further predict the area of the lesion.Keywords
Cite This Article
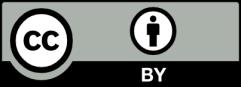
This work is licensed under a Creative Commons Attribution 4.0 International License , which permits unrestricted use, distribution, and reproduction in any medium, provided the original work is properly cited.