Open Access
ARTICLE
Active Learning Strategies for Textual Dataset-Automatic Labelling
1 Department of Computer Science, Sukkur IBA University, Sukkur, 65200, Pakistan
2 Al Quwayiyah College of Sciences and Humanities, Shaqra University, Shaqra, 15526, Saudi Arabia
3 Self-Development Skills Department, Common First Year Deanship, King Saud University, Riyadh, 12373, Saudi Arabia
4 STC’s Artificial Intelligence Chair, Department of Information Systems, College of Computer and Information Sciences,
King Saud University, Riyadh, 11451, Saudi Arabia
5 College of Engineering & Technology, University of Sargodha, Sargodha, 40100, Pakistan
* Corresponding Author: Yazeed Alkhurayyif. Email:
(This article belongs to the Special Issue: Emerging Techniques on Citation Analysis in Scholarly Articles)
Computers, Materials & Continua 2023, 76(2), 1409-1422. https://doi.org/10.32604/cmc.2023.034157
Received 07 July 2022; Accepted 23 September 2022; Issue published 30 August 2023
Abstract
The Internet revolution has resulted in abundant data from various sources, including social media, traditional media, etcetera. Although the availability of data is no longer an issue, data labelling for exploiting it in supervised machine learning is still an expensive process and involves tedious human efforts. The overall purpose of this study is to propose a strategy to automatically label the unlabeled textual data with the support of active learning in combination with deep learning. More specifically, this study assesses the performance of different active learning strategies in automatic labelling of the textual dataset at sentence and document levels. To achieve this objective, different experiments have been performed on the publicly available dataset. In first set of experiments, we randomly choose a subset of instances from training dataset and train a deep neural network to assess performance on test set. In the second set of experiments, we replace the random selection with different active learning strategies to choose a subset of the training dataset to train the same model and reassess its performance on test set. The experimental results suggest that different active learning strategies yield performance improvement of 7% on document level datasets and 3% on sentence level datasets for auto labelling.Keywords
Cite This Article
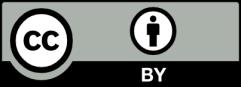