Open Access
ARTICLE
PLDMLT: Multi-Task Learning of Diabetic Retinopathy Using the Pixel-Level Labeled Fundus Images
Department of Computer Science and Engineering, Chongqing University of Technology, Chongqing, 400054, China
* Corresponding Author: Chuncheng Huang. Email:
(This article belongs to the Special Issue: Recent Advances in Ophthalmic Diseases Diagnosis using AI)
Computers, Materials & Continua 2023, 76(2), 1745-1761. https://doi.org/10.32604/cmc.2023.040710
Received 28 March 2023; Accepted 25 May 2023; Issue published 30 August 2023
Abstract
In the field of medical images, pixel-level labels are time-consuming and expensive to acquire, while image-level labels are relatively easier to obtain. Therefore, it makes sense to learn more information (knowledge) from a small number of hard-to-get pixel-level annotated images to apply to different tasks to maximize their usefulness and save time and training costs. In this paper, using Pixel-Level Labeled Images for Multi-Task Learning (PLDMLT), we focus on grading the severity of fundus images for Diabetic Retinopathy (DR). This is because, for the segmentation task, there is a finely labeled mask, while the severity grading task is without classification labels. To this end, we propose a two-stage multi-label learning weakly supervised algorithm, which generates initial classification pseudo labels in the first stage and visualizes heat maps at all levels of severity using Grad-Cam to further provide medical interpretability for the classification task. A multi-task model framework with U-net as the baseline is proposed in the second stage. A label update network is designed to alleviate the gradient balance between the classification and segmentation tasks. Extensive experimental results show that our PLDMLT method significantly outperforms other state-of-the-art methods in DR segmentation on two public datasets, achieving up to 98.897% segmentation accuracy. In addition, our method achieves comparable competitiveness with single-task fully supervised learning in the DR severity grading task.Keywords
Cite This Article
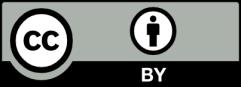
This work is licensed under a Creative Commons Attribution 4.0 International License , which permits unrestricted use, distribution, and reproduction in any medium, provided the original work is properly cited.