Open Access
ARTICLE
Fusion of Feature Ranking Methods for an Effective Intrusion Detection System
1 School of Computer Science and Engineering, VIT-AP University, Andhra Pradesh, India
2 Center of Excellence, AI, and Robotics, VIT-AP University, Andhra Pradesh, India
* Corresponding Author: Seetha Hari. Email:
Computers, Materials & Continua 2023, 76(2), 1721-1744. https://doi.org/10.32604/cmc.2023.040567
Received 23 March 2023; Accepted 19 May 2023; Issue published 30 August 2023
Abstract
Expanding internet-connected services has increased cyberattacks, many of which have grave and disastrous repercussions. An Intrusion Detection System (IDS) plays an essential role in network security since it helps to protect the network from vulnerabilities and attacks. Although extensive research was reported in IDS, detecting novel intrusions with optimal features and reducing false alarm rates are still challenging. Therefore, we developed a novel fusion-based feature importance method to reduce the high dimensional feature space, which helps to identify attacks accurately with less false alarm rate. Initially, to improve training data quality, various preprocessing techniques are utilized. The Adaptive Synthetic oversampling technique generates synthetic samples for minority classes. In the proposed fusion-based feature importance, we use different approaches from the filter, wrapper, and embedded methods like mutual information, random forest importance, permutation importance, Shapley Additive exPlanations (SHAP)-based feature importance, and statistical feature importance methods like the difference of mean and median and standard deviation to rank each feature according to its rank. Then by simple plurality voting, the most optimal features are retrieved. Then the optimal features are fed to various models like Extra Tree (ET), Logistic Regression (LR), Support vector Machine (SVM), Decision Tree (DT), and Extreme Gradient Boosting Machine (XGBM). Then the hyperparameters of classification models are tuned with Halving Random Search cross-validation to enhance the performance. The experiments were carried out on the original imbalanced data and balanced data. The outcomes demonstrate that the balanced data scenario knocked out the imbalanced data. Finally, the experimental analysis proved that our proposed fusion-based feature importance performed well with XGBM giving an accuracy of 99.86%, 99.68%, and 92.4%, with 9, 7 and 8 features by training time of 1.5, 4.5 and 5.5 s on Network Security Laboratory-Knowledge Discovery in Databases (NSL-KDD), Canadian Institute for Cybersecurity (CIC-IDS 2017), and UNSW-NB15, datasets respectively. In addition, the suggested technique has been examined and contrasted with the state of art methods on three datasets.Keywords
Cite This Article
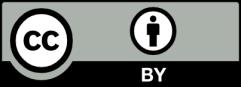