Open Access
ARTICLE
AID4I: An Intrusion Detection Framework for Industrial Internet of Things Using Automated Machine Learning
1 ATASAREN, National Defence University, Istanbul, 34334, Turkey
2 Software In Motion Department, Siemens Advanta, Siemens Corporate Technology, Istanbul, 34870, Turkey
3 Department of Computer Engineering National Defence University, Air Force Academy, Istanbul, 34149, Turkey
* Corresponding Author: Anıl Sezgin. Email:
Computers, Materials & Continua 2023, 76(2), 2121-2143. https://doi.org/10.32604/cmc.2023.040287
Received 12 March 2023; Accepted 09 June 2023; Issue published 30 August 2023
Abstract
By identifying and responding to any malicious behavior that could endanger the system, the Intrusion Detection System (IDS) is crucial for preserving the security of the Industrial Internet of Things (IIoT) network. The benefit of anomaly-based IDS is that they are able to recognize zero-day attacks due to the fact that they do not rely on a signature database to identify abnormal activity. In order to improve control over datasets and the process, this study proposes using an automated machine learning (AutoML) technique to automate the machine learning processes for IDS. Our ground-breaking architecture, known as AID4I, makes use of automatic machine learning methods for intrusion detection. Through automation of preprocessing, feature selection, model selection, and hyperparameter tuning, the objective is to identify an appropriate machine learning model for intrusion detection. Experimental studies demonstrate that the AID4I framework successfully proposes a suitable model. The integrity, security, and confidentiality of data transmitted across the IIoT network can be ensured by automating machine learning processes in the IDS to enhance its capacity to identify and stop threatening activities. With a comprehensive solution that takes advantage of the latest advances in automated machine learning methods to improve network security, AID4I is a powerful and effective instrument for intrusion detection. In preprocessing module, three distinct imputation methods are utilized to handle missing data, ensuring the robustness of the intrusion detection system in the presence of incomplete information. Feature selection module adopts a hybrid approach that combines Shapley values and genetic algorithm. The Parameter Optimization module encompasses a diverse set of 14 classification methods, allowing for thorough exploration and optimization of the parameters associated with each algorithm. By carefully tuning these parameters, the framework enhances its adaptability and accuracy in identifying potential intrusions. Experimental results demonstrate that the AID4I framework can achieve high levels of accuracy in detecting network intrusions up to 14.39% on public datasets, outperforming traditional intrusion detection methods while concurrently reducing the elapsed time for training and testing.Keywords
Cite This Article
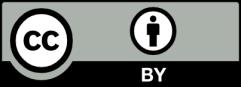