Open Access
ARTICLE
MBB-IoT: Construction and Evaluation of IoT DDoS Traffic Dataset from a New Perspective
1 Department of Artificial Intelligence, People’s Public Security University of China, Beijing, 100038, China
2 Department of Cybersecurity, People’s Public Security University of China, Beijing, 100038, China
* Corresponding Author: Yanhui Du. Email:
Computers, Materials & Continua 2023, 76(2), 2095-2119. https://doi.org/10.32604/cmc.2023.039980
Received 27 February 2023; Accepted 09 June 2023; Issue published 30 August 2023
Abstract
Distributed Denial of Service (DDoS) attacks have always been a major concern in the security field. With the release of malware source codes such as BASHLITE and Mirai, Internet of Things (IoT) devices have become the new source of DDoS attacks against many Internet applications. Although there are many datasets in the field of IoT intrusion detection, such as Bot-IoT, Constrained Application Protocol–Denial of Service (CoAP-DoS), and LATAM-DDoS-IoT (some of the names of DDoS datasets), which mainly focus on DDoS attacks, the datasets describing new IoT DDoS attack scenarios are extremely rare, and only N-BaIoT and IoT-23 datasets used IoT devices as DDoS attackers in the construction process, while they did not use Internet applications as victims either. To supplement the description of the new trend of DDoS attacks in the dataset, we built an IoT environment with mainstream DDoS attack tools such as Mirai and BASHLITE being used to infect IoT devices and implement DDoS attacks against WEB servers. Then, data aggregated into a dataset named MBB-IoT were captured at WEB servers and IoT nodes. After the MBB-IoT dataset was split into a training set and a test set, it was applied to the training and testing of the Random Forests classification algorithm. The multi-class classification metrics were good and all above 90%. Secondly, in a cross-evaluation experiment based on Support Vector Machine (SVM), Light Gradient Boosting Machine (LightGBM), and Long Short Term Memory networks (LSTM) classification algorithms, the training set and test set were derived from different datasets (MBB-IoT or IoT-23), and the test performance is better when MBB-IoT is used as the training set.Keywords
Cite This Article
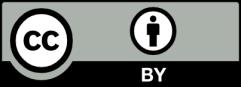
This work is licensed under a Creative Commons Attribution 4.0 International License , which permits unrestricted use, distribution, and reproduction in any medium, provided the original work is properly cited.