Open Access
ARTICLE
Eye-Tracking Based Autism Spectrum Disorder Diagnosis Using Chaotic Butterfly Optimization with Deep Learning Model
1 Department of Computer Science and Engineering, Panimalar Engineering College, Chennai, 600123, India
2 Department of Computer Science, University College of Al Jamoum, Umm Al-Qura University, Makkah, 21421, Saudi Arabia
3 Department of Computer Science and Engineering, Saveetha School of Engineering, Saveetha Institute of Medical and Technical Sciences, Chennai, 602105, India
4 School of Computing, SRM Institute of Science and Technology, Kattankulathur, 603203, India
* Corresponding Author: Surendran Rajendran. Email:
Computers, Materials & Continua 2023, 76(2), 1995-2013. https://doi.org/10.32604/cmc.2023.039644
Received 09 February 2023; Accepted 13 June 2023; Issue published 30 August 2023
Abstract
Autism spectrum disorder (ASD) can be defined as a neurodevelopmental condition or illness that can disturb kids who have heterogeneous characteristics, like changes in behavior, social disabilities, and difficulty communicating with others. Eye tracking (ET) has become a useful method to detect ASD. One vital aspect of moral erudition is the aptitude to have common visual attention. The eye-tracking approach offers valuable data regarding the visual behavior of children for accurate and early detection. Eye-tracking data can offer insightful information about the behavior and thought processes of people with ASD, but it is important to be aware of its limitations and to combine it with other types of data and assessment techniques to increase the precision of ASD detection. It operates by scanning the paths of eyes for extracting a series of eye projection points on images for examining the behavior of children with autism. The purpose of this research is to use deep learning to identify autistic disorders based on eye tracking. The Chaotic Butterfly Optimization technique is used to identify this specific disturbance. Therefore, this study develops an ET-based Autism Spectrum Disorder Diagnosis using Chaotic Butterfly Optimization with Deep Learning (ETASD-CBODL) technique. The presented ETASD-CBODL technique mainly focuses on the recognition of ASD via the ET and DL models. To accomplish this, the ETASD-CBODL technique exploits the U-Net segmentation technique to recognize interested AREASS. In addition, the ETASD-CBODL technique employs Inception v3 feature extraction with CBO algorithm-based hyperparameter optimization. Finally, the long-short-term memory (LSTM) model is exploited for the recognition and classification of ASD. To assess the performance of the ETASD-CBODL technique, a series of simulations were performed on datasets from the figure-shared data repository. The experimental values of accuracy (99.29%), precision (98.78%), sensitivity (99.29%) and specificity (99.29%) showed a better performance in the ETASD-CBODL technique over recent approaches.Keywords
Cite This Article
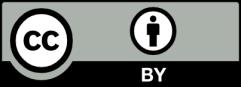
This work is licensed under a Creative Commons Attribution 4.0 International License , which permits unrestricted use, distribution, and reproduction in any medium, provided the original work is properly cited.