Open Access
ARTICLE
Relevant Visual Semantic Context-Aware Attention-Based Dialog
1 National Advanced IPv6 Centre, Universiti Sains Malaysia, Penang, Malaysia
2 Lee Kong Chian Faculty of Engineering and Science (LKCFES), Universiti Tunku Abdul Rahman, Sungai Long, Selangor, Malaysia
* Corresponding Author: Yung-Wey Chong. Email:
Computers, Materials & Continua 2023, 76(2), 2337-2354. https://doi.org/10.32604/cmc.2023.038695
Received 25 December 2022; Accepted 23 May 2023; Issue published 30 August 2023
Abstract
The existing dataset for visual dialog comprises multiple rounds of questions and a diverse range of image contents. However, it faces challenges in overcoming visual semantic limitations, particularly in obtaining sufficient context from visual and textual aspects of images. This paper proposes a new visual dialog dataset called Diverse History-Dialog (DS-Dialog) to address the visual semantic limitations faced by the existing dataset. DS-Dialog groups relevant histories based on their respective Microsoft Common Objects in Context (MSCOCO) image categories and consolidates them for each image. Specifically, each MSCOCO image category consists of top relevant histories extracted based on their semantic relationships between the original image caption and historical context. These relevant histories are consolidated for each image, and DS-Dialog enhances the current dataset by adding new context-aware relevant history to provide more visual semantic context for each image. The new dataset is generated through several stages, including image semantic feature extraction, keyphrase extraction, relevant question extraction, and relevant history dialog generation. The DS-Dialog dataset contains about 2.6 million question-answer pairs, where 1.3 million pairs correspond to existing VisDial’s question-answer pairs, and the remaining 1.3 million pairs include a maximum of 5 image features for each VisDial image, with each image comprising 10-round relevant question-answer pairs. Moreover, a novel adaptive relevant history selection is proposed to resolve missing visual semantic information for each image. DS-Dialog is used to benchmark the performance of previous visual dialog models and achieves better performance than previous models. Specifically, the proposed DS-Dialog model achieves an 8% higher mean reciprocal rank (MRR), 11% higher R@1%, 6% higher R@5%, 5% higher R@10%, and 8% higher normalized discounted cumulative gain (NDCG) compared to LF. DS-Dialog also achieves approximately 1 point improvement on R@k, mean, MRR, and NDCG compared to the original RVA, and 2 points improvement compared to LF and DualVD. These results demonstrates the importance of the relevant semantic historical context in enhancing the visual semantic relationship between textual and visual representations of the images and questions.Keywords
Cite This Article
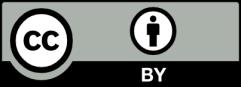
This work is licensed under a Creative Commons Attribution 4.0 International License , which permits unrestricted use, distribution, and reproduction in any medium, provided the original work is properly cited.