Open Access
ARTICLE
Developing a Breast Cancer Resistance Protein Substrate Prediction System Using Deep Features and LDA
1 Department of Computer Science, Air University, Sector E-9, PAF Complex, Islamabad, 44000, Pakistan
2 Department of ICT Convergence System Engineering, Chonnam National University, Gwangju, Korea
3 Directorate of National Repository, Islamabad, Pakistan
4 Department of Computer Science, Bahauddin Zakariya University, Multan, 60000, Pakistan
5 Department of Computer Science and Information, College of Science in Zulfi, Majmaah University, Al- Majmaah, 11952, Saudi Arabia
6 Pakistan Atomic Energy Commission, General Hospital, Islamabad, Pakistan
* Corresponding Author: Jin Young Kim. Email:
(This article belongs to the Special Issue: Intelligent Computational Models based on Machine Learning and Deep Learning for Diagnosis System)
Computers, Materials & Continua 2023, 76(2), 1643-1663. https://doi.org/10.32604/cmc.2023.038578
Received 19 December 2022; Accepted 10 April 2023; Issue published 30 August 2023
Abstract
Breast cancer resistance protein (BCRP) is an important resistance protein that significantly impacts anticancer drug discovery, treatment, and rehabilitation. Early identification of BCRP substrates is quite a challenging task. This study aims to predict early substrate structure, which can help to optimize anticancer drug development and clinical diagnosis. For this study, a novel intelligent approach-based methodology is developed by modifying the ResNet101 model using transfer learning (TL) for automatic deep feature (DF) extraction followed by classification with linear discriminant analysis algorithm (TLRNDF-LDA). This study utilized structural fingerprints, which are exploited by DF contrary to conventional molecular descriptors. The proposed in silico model achieved an outstanding accuracy performance of 98.56% on test data compared to other state-of-the-art approaches using standard quality measures. Furthermore, the model’s efficacy is validated via a statistical analysis ANOVA test. It is demonstrated that the developed model can be used effectively for early prediction of the substrate structure. The pipeline of this study is flexible and can be extended for in vitro assessment efficacy of anticancer drug response, identification of BCRP functions in transport experiments, and prediction of prostate or lung cancer cell lines.Keywords
Cite This Article
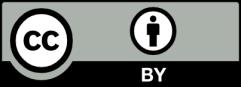