Open Access
ARTICLE
A Machine Learning-Based Distributed Denial of Service Detection Approach for Early Warning in Internet Exchange Points
School of Technology and Innovation College of Business, Innovation, Leadership, and Technology (BILT), Marymount University, Arlington, Virginia, 22207, USA
* Corresponding Author: Salem Alhayani. Email:
Computers, Materials & Continua 2023, 76(2), 2235-2259. https://doi.org/10.32604/cmc.2023.038003
Received 24 November 2022; Accepted 24 May 2023; Issue published 30 August 2023
Abstract
The Internet service provider (ISP) is the heart of any country’s Internet infrastructure and plays an important role in connecting to the World Wide Web. Internet exchange point (IXP) allows the interconnection of two or more separate network infrastructures. All Internet traffic entering a country should pass through its IXP. Thus, it is an ideal location for performing malicious traffic analysis. Distributed denial of service (DDoS) attacks are becoming a more serious daily threat. Malicious actors in DDoS attacks control numerous infected machines known as botnets. Botnets are used to send numerous fake requests to overwhelm the resources of victims and make them unavailable for some periods. To date, such attacks present a major devastating security threat on the Internet. This paper proposes an effective and efficient machine learning (ML)-based DDoS detection approach for the early warning and protection of the Saudi Arabia Internet exchange point (SAIXP) platform. The effectiveness and efficiency of the proposed approach are verified by selecting an accurate ML method with a small number of input features. A chi-square method is used for feature selection because it is easier to compute than other methods, and it does not require any assumption about feature distribution values. Several ML methods are assessed using holdout and 10-fold tests on a public large-size dataset. The experiments showed that the performance of the decision tree (DT) classifier achieved a high accuracy result (99.98%) with a small number of features (10 features). The experimental results confirm the applicability of using DT and chi-square for DDoS detection and early warning in SAIXP.Keywords
Cite This Article
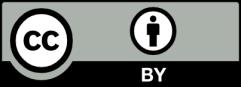