Open Access
ARTICLE
Context Awareness by Noise-Pattern Analysis of a Smart Factory
1 Department of Software Convergence, Soonchunhyang University, Asan, 31538, Korea
2 Department of Computer Software Engineering, Soonchunhyang University, Asan, 31538, Korea
* Corresponding Author: Dae-Young Kim. Email:
Computers, Materials & Continua 2023, 76(2), 1497-1514. https://doi.org/10.32604/cmc.2023.034914
Received 01 August 2022; Accepted 04 December 2022; Issue published 30 August 2023
Abstract
Recently, to build a smart factory, research has been conducted to perform fault diagnosis and defect detection based on vibration and noise signals generated when a mechanical system is driven using deep-learning technology, a field of artificial intelligence. Most of the related studies apply various audio-feature extraction techniques to one-dimensional raw data to extract sound-specific features and then classify the sound by using the derived spectral image as a training dataset. However, compared to numerical raw data, learning based on image data has the disadvantage that creating a training dataset is very time-consuming. Therefore, we devised a two-step data preprocessing method that efficiently detects machine anomalies in numerical raw data. In the first preprocessing process, sound signal information is analyzed to extract features, and in the second preprocessing process, data filtering is performed by applying the proposed algorithm. An efficient dataset was built for model learning through a total of two steps of data preprocessing. In addition, both showed excellent performance in the training accuracy of the model that entered each dataset, but it can be seen that the time required to build the dataset was 203 s compared to 39 s, which is about 5.2 times than when building the image dataset.Keywords
Cite This Article
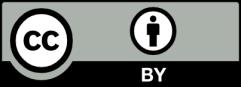