Open Access
ARTICLE
Modified Dragonfly Optimization with Machine Learning Based Arabic Text Recognition
1 Department of Language Preparation, Arabic Language Teaching Institute, Princess Nourah bint Abdulrahman University, P.O. Box 84428, Riyadh, 11671, Saudi Arabia
2 Prince Saud AlFaisal Institute for Diplomatic Studies, Riyadh, Saudi Arabia
3 Department of Industrial Engineering, College of Engineering at Alqunfudah, Umm Al-Qura University, Makkah, Saudi Arabia
4 Department of Computer Science, College of Computing and Information Technology, Shaqra University, Shaqra, Saudi Arabia
5 Department of Information Systems, College of Computer and Information Sciences, Princess Nourah bint Abdulrahman University, P.O. Box 84428, Riyadh, 11671, Saudi Arabia
6 Department of Computer Science, Faculty of Computers and Information Technology, Future University in Egypt, New Cairo, 11835, Egypt
7 Department of Computer and Self Development, Preparatory Year Deanship, Prince Sattam bin Abdulaziz University, AlKharj, Saudi Arabia
* Corresponding Author: Abdelwahed Motwakel. Email:
Computers, Materials & Continua 2023, 76(2), 1537-1554. https://doi.org/10.32604/cmc.2023.034196
Received 08 July 2022; Accepted 27 August 2022; Issue published 30 August 2023
Abstract
Text classification or categorization is the procedure of automatically tagging a textual document with most related labels or classes. When the number of labels is limited to one, the task becomes single-label text categorization. The Arabic texts include unstructured information also like English texts, and that is understandable for machine learning (ML) techniques, the text is changed and demonstrated by numerical value. In recent times, the dominant method for natural language processing (NLP) tasks is recurrent neural network (RNN), in general, long short term memory (LSTM) and convolutional neural network (CNN). Deep learning (DL) models are currently presented for deriving a massive amount of text deep features to an optimum performance from distinct domains such as text detection, medical image analysis, and so on. This paper introduces a Modified Dragonfly Optimization with Extreme Learning Machine for Text Representation and Recognition (MDFO-EMTRR) model on Arabic Corpus. The presented MDFO-EMTRR technique mainly concentrates on the recognition and classification of the Arabic text. To achieve this, the MDFO-EMTRR technique encompasses data pre-processing to transform the input data into compatible format. Next, the ELM model is utilized for the representation and recognition of the Arabic text. At last, the MDFO algorithm was exploited for optimal tuning of the parameters related to the ELM method and thereby accomplish enhanced classifier results. The experimental result analysis of the MDFO-EMTRR system was performed on benchmark datasets and attained maximum accuracy of 99.74%.Keywords
Cite This Article
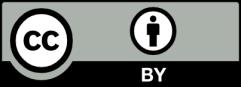
This work is licensed under a Creative Commons Attribution 4.0 International License , which permits unrestricted use, distribution, and reproduction in any medium, provided the original work is properly cited.