Open Access
ARTICLE
Early Diagnosis of Lung Tumors for Extending Patients’ Life Using Deep Neural Networks
1 Department of Computer Science and Engineering, SRM Institute of Science and Technology, Ramapuram, Chennai, India
2 Department of Computer Science and Engineering, Saveetha Engineering College, Chennai, India
3 Department of Computer Science and Engineering, Vardhaman College of Engineering, Hyderabad, India
4 Department of Computer Science and Information Engineering, National Yunlin University of Science and Technology, Yunlin 64002, Taiwan
5 Intelligence Recognition Industry Service Research Center, National Yunlin University of Science and Technology, Yunlin 64002, Taiwan
6 Chitkara University Institute of Engineering and Technology, Chitkara University, Punjab, India
7 Department of Computing Science, University of Aberdeen, Aberdeen, UK
8 Software Engineering Department, College of Computer and Information Sciences, King Saud University, Riyadh 12372, Saudi Arabia
9 Computer Science Department, Community College, King Saud University, Riyadh 11437, Saudi Arabia
10 School of Computer & Communication Engineering, Changsha University of Science & Technology, Changsha, China
* Corresponding Author: Shih-Yu Chen. Email:
Computers, Materials & Continua 2023, 76(1), 993-1007. https://doi.org/10.32604/cmc.2023.039567
Received 06 February 2023; Accepted 23 April 2023; Issue published 08 June 2023
Abstract
The medical community has more concern on lung cancer analysis. Medical experts’ physical segmentation of lung cancers is time-consuming and needs to be automated. The research study’s objective is to diagnose lung tumors at an early stage to extend the life of humans using deep learning techniques. Computer-Aided Diagnostic (CAD) system aids in the diagnosis and shortens the time necessary to detect the tumor detected. The application of Deep Neural Networks (DNN) has also been exhibited as an excellent and effective method in classification and segmentation tasks. This research aims to separate lung cancers from images of Magnetic Resonance Imaging (MRI) with threshold segmentation. The Honey hook process categorizes lung cancer based on characteristics retrieved using several classifiers. Considering this principle, the work presents a solution for image compression utilizing a Deep Wave Auto-Encoder (DWAE). The combination of the two approaches significantly reduces the overall size of the feature set required for any future classification process performed using DNN. The proposed DWAE-DNN image classifier is applied to a lung imaging dataset with Radial Basis Function (RBF) classifier. The study reported promising results with an accuracy of 97.34%, whereas using the Decision Tree (DT) classifier has an accuracy of 94.24%. The proposed approach (DWAE-DNN) is found to classify the images with an accuracy of 98.67%, either as malignant or normal patients. In contrast to the accuracy requirements, the work also uses the benchmark standards like specificity, sensitivity, and precision to evaluate the efficiency of the network. It is found from an investigation that the DT classifier provides the maximum performance in the DWAE-DNN depending on the network’s performance on image testing, as shown by the data acquired by the categorizers themselves.Keywords
Cite This Article
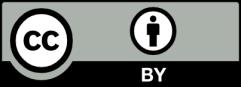
This work is licensed under a Creative Commons Attribution 4.0 International License , which permits unrestricted use, distribution, and reproduction in any medium, provided the original work is properly cited.