Open Access
ARTICLE
Spatial Correlation Module for Classification of Multi-Label Ocular Diseases Using Color Fundus Images
1 Department of Software Engineering, Faculty of Computer Sciences, Lahore Garrison University, Lahore, Pakistan
2 Department of Computer Science, School of Systems and Technology, University of Management and Technology, Lahore, 54000, Pakistan
3 Department of Computer Science & Information Technology, University of Engineering and Technology (UET), Peshawar, Pakistan
4 Department of Computer Science, TIMES Institute, Multan, 60000, Pakistan
5 Department of Software Engineering, School of Systems and Technology, University of Management and Technology, Lahore, 54000, Pakistan
* Corresponding Authors: Ali Haider Khan. Email: ,
(This article belongs to the Special Issue: Recent Advances in Ophthalmic Diseases Diagnosis using AI)
Computers, Materials & Continua 2023, 76(1), 133-150. https://doi.org/10.32604/cmc.2023.039518
Received 02 February 2023; Accepted 11 April 2023; Issue published 08 June 2023
Abstract
To prevent irreversible damage to one’s eyesight, ocular diseases (ODs) need to be recognized and treated immediately. Color fundus imaging (CFI) is a screening technology that is both effective and economical. According to CFIs, the early stages of the disease are characterized by a paucity of observable symptoms, which necessitates the prompt creation of automated and robust diagnostic algorithms. The traditional research focuses on image-level diagnostics that attend to the left and right eyes in isolation without making use of pertinent correlation data between the two sets of eyes. In addition, they usually only target one or a few different kinds of eye diseases at the same time. In this study, we design a patient-level multi-label OD (PLML_ODs) classification model that is based on a spatial correlation network (SCNet). This model takes into consideration the relevance of patient-level diagnosis combining bilateral eyes and multi-label ODs classification. PLML_ODs is made up of three parts: a backbone convolutional neural network (CNN) for feature extraction i.e., DenseNet-169, a SCNet for feature correlation, and a classifier for the development of classification scores. The DenseNet-169 is responsible for retrieving two separate sets of attributes, one from each of the left and right CFI. After then, the SCNet will record the correlations between the two feature sets on a pixel-by-pixel basis. After the attributes have been analyzed, they are integrated to provide a representation at the patient level. Throughout the whole process of ODs categorization, the patient-level representation will be used. The efficacy of the PLML_ODs is examined using a soft margin loss on a dataset that is readily accessible to the public, and the results reveal that the classification performance is significantly improved when compared to several baseline approaches.Keywords
Cite This Article
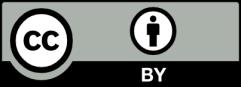