Open Access
ARTICLE
An Improved Fully Automated Breast Cancer Detection and Classification System
1 Department of Electrical Engineering, Faculty of Engineering at Rabigh, King Abdulaziz University, Jeddah, Saudi Arabia
2 Department of Electrical Engineering, College of Engineering, Northern Border University, Arar, Saudi Arabia
* Corresponding Author: Ahmed A. Alsheikhy. Email:
(This article belongs to the Special Issue: Smart Solutions to Develop New Technologies for Healthcare)
Computers, Materials & Continua 2023, 76(1), 731-751. https://doi.org/10.32604/cmc.2023.039433
Received 29 January 2023; Accepted 20 April 2023; Issue published 08 June 2023
Abstract
More than 500,000 patients are diagnosed with breast cancer annually. Authorities worldwide reported a death rate of 11.6% in 2018. Breast tumors are considered a fatal disease and primarily affect middle-aged women. Various approaches to identify and classify the disease using different technologies, such as deep learning and image segmentation, have been developed. Some of these methods reach 99% accuracy. However, boosting accuracy remains highly important as patients’ lives depend on early diagnosis and specified treatment plans. This paper presents a fully computerized method to detect and categorize tumor masses in the breast using two deep-learning models and a classifier on different datasets. This method specifically uses ResNet50 and AlexNet, convolutional neural networks (CNNs), for deep learning and a K-Nearest-Neighbor (KNN) algorithm to classify data. Various experiments have been conducted on five datasets: the Mammographic Image Analysis Society (MIAS), Breast Cancer Histopathological Annotation and Diagnosis (BreCaHAD), King Abdulaziz University Breast Cancer Mammogram Dataset (KAU-BCMD), Breast Histopathology Images (BHI), and Breast Cancer Histopathological Image Classification (BreakHis). These datasets were used to train, validate, and test the presented method. The obtained results achieved an average of 99.38% accuracy, surpassing other models. Essential performance quantities, including precision, recall, specificity, and F-score, reached 99.71%, 99.46%, 98.08%, and 99.67%, respectively. These outcomes indicate that the presented method offers essential aid to pathologists diagnosing breast cancer. This study suggests using the implemented algorithm to support physicians in analyzing breast cancer correctly.Keywords
Cite This Article
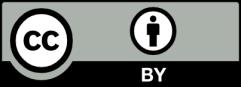
This work is licensed under a Creative Commons Attribution 4.0 International License , which permits unrestricted use, distribution, and reproduction in any medium, provided the original work is properly cited.