Open Access
ARTICLE
ECGAN: Translate Real World to Cartoon Style Using Enhanced Cartoon Generative Adversarial Network
Department of Cooperative Course of Performance, Film & Animation, Sejong University, Seoul, 05006, Korea
* Corresponding Author: Yixin Tang. Email:
Computers, Materials & Continua 2023, 76(1), 1195-1212. https://doi.org/10.32604/cmc.2023.039182
Received 13 January 2023; Accepted 10 April 2023; Issue published 08 June 2023
Abstract
Visual illustration transformation from real-world to cartoon images is one of the famous and challenging tasks in computer vision. Image-to-image translation from real-world to cartoon domains poses issues such as a lack of paired training samples, lack of good image translation, low feature extraction from the previous domain images, and lack of high-quality image translation from the traditional generator algorithms. To solve the above-mentioned issues, paired independent model, high-quality dataset, Bayesian-based feature extractor, and an improved generator must be proposed. In this study, we propose a high-quality dataset to reduce the effect of paired training samples on the model’s performance. We use a Bayesian Very Deep Convolutional Network (VGG)-based feature extractor to improve the performance of the standard feature extractor because Bayesian inference regularizes weights well. The generator from the Cartoon Generative Adversarial Network (GAN) is modified by introducing a depthwise convolution layer and channel attention mechanism to improve the performance of the original generator. We have used the Fréchet inception distance (FID) score and user preference score to evaluate the performance of the model. The FID scores obtained for the generated cartoon and real-world images are 107 and 76 for the TCC style, and 137 and 57 for the Hayao style, respectively. User preference score is also calculated to evaluate the quality of generated images and our proposed model acquired a high preference score compared to other models. We achieved stunning results in producing high-quality cartoon images, demonstrating the proposed model’s effectiveness in transferring style between authentic images and cartoon images.Keywords
Cite This Article
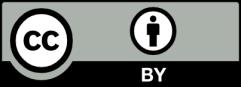
This work is licensed under a Creative Commons Attribution 4.0 International License , which permits unrestricted use, distribution, and reproduction in any medium, provided the original work is properly cited.