Open Access
ARTICLE
Modeling Price-Aware Session-Based Recommendation Based on Graph Neural Network
College of Computer Science & Technology, Xi’an University of Science and Technology, Xi’an, 710600, China
* Corresponding Author: Jian Feng. Email:
Computers, Materials & Continua 2023, 76(1), 397-413. https://doi.org/10.32604/cmc.2023.038741
Received 27 December 2022; Accepted 17 April 2023; Issue published 08 June 2023
Abstract
Session-based Recommendation (SBR) aims to accurately recommend a list of items to users based on anonymous historical session sequences. Existing methods for SBR suffer from several limitations: SBR based on Graph Neural Network often has information loss when constructing session graphs; Inadequate consideration is given to influencing factors, such as item price, and users’ dynamic interest evolution is not taken into account. A new session recommendation model called Price-aware Session-based Recommendation (PASBR) is proposed to address these limitations. PASBR constructs session graphs by information lossless approaches to fully encode the original session information, then introduces item price as a new factor and models users’ price tolerance for various items to influence users’ preferences. In addition, PASBR proposes a new method to encode user intent at the item category level and tries to capture the dynamic interest of users over time. Finally, PASBR fuses the multi-perspective features to generate the global representation of users and make a prediction. Specifically, the intent, the short-term and long-term interests, and the dynamic interests of a user are combined. Experiments on two real-world datasets show that PASBR can outperform representative baselines for SBR.Keywords
Cite This Article
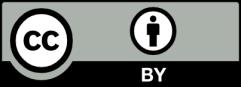