Open Access
ARTICLE
Analyzing Arabic Twitter-Based Patient Experience Sentiments Using Multi-Dialect Arabic Bidirectional Encoder Representations from Transformers
Department of Computer Science, College of Computer and Information Sciences, King Saud University, P.O. Box 266, Riyadh 11362, Saudi Arabia
* Corresponding Author: Sarab AlMuhaideb. Email:
(This article belongs to the Special Issue: Advance Machine Learning for Sentiment Analysis over Various Domains and Applications)
Computers, Materials & Continua 2023, 76(1), 195-220. https://doi.org/10.32604/cmc.2023.038368
Received 09 December 2022; Accepted 10 April 2023; Issue published 08 June 2023
Abstract
Healthcare organizations rely on patients’ feedback and experiences to evaluate their performance and services, thereby allowing such organizations to improve inadequate services and address any shortcomings. According to the literature, social networks and particularly Twitter are effective platforms for gathering public opinions. Moreover, recent studies have used natural language processing to measure sentiments in text segments collected from Twitter to capture public opinions about various sectors, including healthcare. The present study aimed to analyze Arabic Twitter-based patient experience sentiments and to introduce an Arabic patient experience corpus. The authors collected 12,400 tweets from Arabic patients discussing patient experiences related to healthcare organizations in Saudi Arabia from 1 January 2008 to 29 January 2022. The tweets were labeled according to sentiment (positive or negative) and sector (public or private), and thereby the Hospital Patient Experiences in Saudi Arabia (HoPE-SA) dataset was produced. A simple statistical analysis was conducted to examine differences in patient views of healthcare sectors. The authors trained five models to distinguish sentiments in tweets automatically with the following schemes: a transformer-based model fine-tuned with deep learning architecture and a transformer-based model fine-tuned with simple architecture, using two different transformer-based embeddings based on Bidirectional Encoder Representations from Transformers (BERT), Multi-dialect Arabic BERT (MARBERT), and multilingual BERT (mBERT), as well as a pre-trained word2vec model with a support vector machine classifier. This is the first study to investigate the use of a bidirectional long short-term memory layer followed by a feedforward neural network for the fine-tuning of MARBERT. The deep-learning fine-tuned MARBERT-based model—the authors’ best-performing model—achieved accuracy, micro-F1, and macro-F1 scores of 98.71%, 98.73%, and 98.63%, respectively.Keywords
Cite This Article
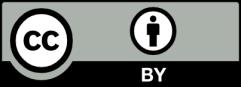