Open Access
ARTICLE
Leveraging Gradient-Based Optimizer and Deep Learning for Automated Soil Classification Model
1 Department of Information Systems, College of Computer and Information Sciences, Princess Nourah bint Abdulrahman University, P. O. Box 84428, Riyadh, 11671, Saudi Arabia
2 Department of Computer and Self Development, Preparatory Year Deanship, Prince Sattam bin Abdulaziz University, AlKharj, Saudi Arabia
3 Department of Software Engineering, College of Computer and Information Sciences, King Saud University, P.O. box 103786, Riyadh, 11543, Saudi Arabia
4 Department of Computer Science, Faculty of Computers and Information Technology, Future University in Egypt, New Cairo, 11835, Egypt
* Corresponding Author: Mohammed Rizwanullah. Email:
Computers, Materials & Continua 2023, 76(1), 975-992. https://doi.org/10.32604/cmc.2023.037936
Received 22 November 2022; Accepted 11 April 2023; Issue published 08 June 2023
Abstract
Soil classification is one of the emanating topics and major concerns in many countries. As the population has been increasing at a rapid pace, the demand for food also increases dynamically. Common approaches used by agriculturalists are inadequate to satisfy the rising demand, and thus they have hindered soil cultivation. There comes a demand for computer-related soil classification methods to support agriculturalists. This study introduces a Gradient-Based Optimizer and Deep Learning (DL) for Automated Soil Classification (GBODL-ASC) technique. The presented GBODL-ASC technique identifies various kinds of soil using DL and computer vision approaches. In the presented GBODL-ASC technique, three major processes are involved. At the initial stage, the presented GBODL-ASC technique applies the GBO algorithm with the EfficientNet prototype to generate feature vectors. For soil categorization, the GBODL-ASC procedure uses an arithmetic optimization algorithm (AOA) with a Back Propagation Neural Network (BPNN) model. The design of GBO and AOA algorithms assist in the proper selection of parameter values for the EfficientNet and BPNN models, respectively. To demonstrate the significant soil classification outcomes of the GBODL-ASC methodology, a wide-ranging simulation analysis is performed on a soil dataset comprising 156 images and five classes. The simulation values show the betterment of the GBODL-ASC model through other models with maximum precision of 95.64%.Keywords
Cite This Article
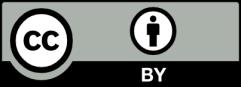