Open Access
ARTICLE
Plant Leaf Diseases Classification Using Improved K-Means Clustering and SVM Algorithm for Segmentation
1 Department of Computer Sciences, College of Computer and Information Sciences, Princess Nourah Bint Abdulrahman University, Riyadh, Saudi Arabia
2 Department of Computer Science, Faculty of Computers and Information, South Valley University, Qena, 83523, Egypt
3 Faculty of Science, New Valley University, El-Kharga, 72511, Egypt
4 Department of Computer Science, Faculty of Computer and Information Sciences, Ain Shams University, Cairo, 11566, Egypt
* Corresponding Author: Hussein Abulkasim. Email:
Computers, Materials & Continua 2023, 76(1), 367-382. https://doi.org/10.32604/cmc.2023.037310
Received 30 October 2022; Accepted 12 April 2023; Issue published 08 June 2023
Abstract
Several pests feed on leaves, stems, bases, and the entire plant, causing plant illnesses. As a result, it is vital to identify and eliminate the disease before causing any damage to plants. Manually detecting plant disease and treating it is pretty challenging in this period. Image processing is employed to detect plant disease since it requires much effort and an extended processing period. The main goal of this study is to discover the disease that affects the plants by creating an image processing system that can recognize and classify four different forms of plant diseases, including Phytophthora infestans, Fusarium graminearum, Puccinia graminis, tomato yellow leaf curl. Therefore, this work uses the Support vector machine (SVM) classifier to detect and classify the plant disease using various steps like image acquisition, Pre-processing, Segmentation, feature extraction, and classification. The gray level co-occurrence matrix (GLCM) and the local binary pattern features (LBP) are used to identify the disease-affected portion of the plant leaf. According to experimental data, the proposed technology can correctly detect and diagnose plant sickness with a 97.2 percent accuracy.Keywords
Cite This Article
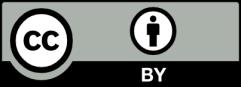