Open Access
ARTICLE
A Double-Compensation-Based Federated Learning Scheme for Data Privacy Protection in a Social IoT Scenario
1 School of Artificial Intelligence, Beijing Normal University, Beijing, 100875, China
2 Engineering Research Center of Intelligent Technology and Educational Application, Ministry of Education, Beijing, 100875, China
* Corresponding Author: Qingyun Xiong. Email:
(This article belongs to the Special Issue: Recent Advances on Security and Privacy of Multimedia Big Data in the Social Internet of Things)
Computers, Materials & Continua 2023, 76(1), 827-848. https://doi.org/10.32604/cmc.2023.036450
Received 30 September 2022; Accepted 06 April 2023; Issue published 08 June 2023
Abstract
Nowadays, smart wearable devices are used widely in the Social Internet of Things (IoT), which record human physiological data in real time. To protect the data privacy of smart devices, researchers pay more attention to federated learning. Although the data leakage problem is somewhat solved, a new challenge has emerged. Asynchronous federated learning shortens the convergence time, while it has time delay and data heterogeneity problems. Both of the two problems harm the accuracy. To overcome these issues, we propose an asynchronous federated learning scheme based on double compensation to solve the problem of time delay and data heterogeneity problems. The scheme improves the Delay Compensated Asynchronous Stochastic Gradient Descent (DC-ASGD) algorithm based on the second-order Taylor expansion as the delay compensation. It adds the FedProx operator to the objective function as the heterogeneity compensation. Besides, the proposed scheme motivates the federated learning process by adjusting the importance of the participants and the central server. We conduct multiple sets of experiments in both conventional and heterogeneous scenarios. The experimental results show that our scheme improves the accuracy by about 5% while keeping the complexity constant. We can find that our scheme converges more smoothly during training and adapts better in heterogeneous environments through numerical experiments. The proposed double-compensation-based federated learning scheme is highly accurate, flexible in terms of participants and smooth the training process. Hence it is deemed suitable for data privacy protection of smart wearable devices.Keywords
Cite This Article
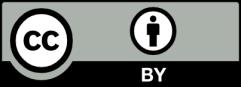
This work is licensed under a Creative Commons Attribution 4.0 International License , which permits unrestricted use, distribution, and reproduction in any medium, provided the original work is properly cited.