Open Access
ARTICLE
Virtual Machine Consolidation with Multi-Step Prediction and Affinity-Aware Technique for Energy-Efficient Cloud Data Centers
School of Cyber Science and Engineering, Southeast University, Nanjing, 211189, China
* Corresponding Author: Pingping Li. Email:
Computers, Materials & Continua 2023, 76(1), 81-105. https://doi.org/10.32604/cmc.2023.039076
Received 09 January 2023; Accepted 10 April 2023; Issue published 08 June 2023
Abstract
Virtual machine (VM) consolidation is an effective way to improve resource utilization and reduce energy consumption in cloud data centers. Most existing studies have considered VM consolidation as a bin-packing problem, but the current schemes commonly ignore the long-term relationship between VMs and hosts. In addition, there is a lack of long-term consideration for resource optimization in the VM consolidation, which results in unnecessary VM migration and increased energy consumption. To address these limitations, a VM consolidation method based on multi-step prediction and affinity-aware technique for energy-efficient cloud data centers (MPaAFVMC) is proposed. The proposed method uses an improved linear regression prediction algorithm to predict the next-moment resource utilization of hosts and VMs, and obtains the stage demand of resources in the future period through multi-step prediction, which is realized by iterative prediction. Then, based on the multi-step prediction, an affinity model between the VM and host is designed using the first-order correlation coefficient and Euclidean distance. During the VM consolidation, the affinity value is used to select the migration VM and placement host. The proposed method is compared with the existing consolidation algorithms on the PlanetLab and Google cluster real workload data using the CloudSim simulation platform. Experimental results show that the proposed method can achieve significant improvement in reducing energy consumption, VM migration costs, and service level agreement (SLA) violations.Keywords
Cite This Article
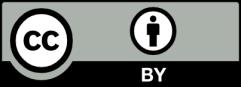
This work is licensed under a Creative Commons Attribution 4.0 International License , which permits unrestricted use, distribution, and reproduction in any medium, provided the original work is properly cited.