Open Access
ARTICLE
Image Generation of Tomato Leaf Disease Identification Based on Small-ACGAN
1 School of Mathematics and Computer Science, Zhejiang A&F University, Hangzhou, 311300, China
2 Zhejiang Provincial Key Laboratory of Forestry Intelligent Monitoring and Information Technology Research,
Hangzhou, 311300, China
3 School of Humanities and Law, Zhejiang A&F University, Hangzhou, 311300, China
4 Department of Computer Science and Engineering, Lehigh University, PA, 18015, USA
* Corresponding Author: Mengjun Tong. Email:
Computers, Materials & Continua 2023, 76(1), 175-194. https://doi.org/10.32604/cmc.2023.037342
Received 31 October 2022; Accepted 07 March 2023; Issue published 08 June 2023
Abstract
Plant diseases have become a challenging threat in the agricultural field. Various learning approaches for plant disease detection and classification have been adopted to detect and diagnose these diseases early. However, deep learning entails extensive data for training, and it may be challenging to collect plant datasets. Even though plant datasets can be collected, they may be uneven in quantity. As a result, the problem of classification model overfitting arises. This study targets this issue and proposes an auxiliary classifier GAN (small-ACGAN) model based on a small number of datasets to extend the available data. First, after comparing various attention mechanisms, this paper chose to add the lightweight Coordinate Attention (CA) to the generator module of Auxiliary Classifier GANs (ACGAN) to improve the image quality. Then, a gradient penalty mechanism was added to the loss function to improve the training stability of the model. Experiments show that the proposed method can best improve the recognition accuracy of the classifier with the doubled dataset. On AlexNet, the accuracy was increased by 11.2%. In addition, small-ACGAN outperformed the other three GANs used in the experiment. Moreover, the experimental accuracy, precision, recall, and F1 scores of the five convolutional neural network (CNN) classifiers on the enhanced dataset improved by an average of 3.74%, 3.48%, 3.74%, and 3.80% compared to the original dataset. Furthermore, the accuracy of MobileNetV3 reached 97.9%, which fully demonstrated the feasibility of this approach. The general experimental results indicate that the method proposed in this paper provides a new dataset expansion method for effectively improving the identification accuracy and can play an essential role in expanding the dataset of the sparse number of plant diseases.Keywords
Cite This Article
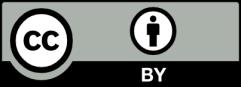
This work is licensed under a Creative Commons Attribution 4.0 International License , which permits unrestricted use, distribution, and reproduction in any medium, provided the original work is properly cited.