Open Access
ARTICLE
Strategy for Rapid Diabetic Retinopathy Exposure Based on Enhanced Feature Extraction Processing
1 Department of Computer Science and Business Systems, M. Kumarasamy College of Engineering, Karur, 639113, Tamilnadu, India
2 Department of Information Technology, Saveetha School of Engineering, Saveetha Institute of Medical and Technical Sciences, SIMATS, Chennai, 602117, Tamil Nadu, India
* Corresponding Author: V. Banupriya. Email:
Computers, Materials & Continua 2023, 75(3), 5597-5613. https://doi.org/10.32604/cmc.2023.038696
Received 25 December 2022; Accepted 07 March 2023; Issue published 29 April 2023
Abstract
In the modern world, one of the most severe eye infections brought on by diabetes is known as diabetic retinopathy (DR), which will result in retinal damage, and, thus, lead to blindness. Diabetic retinopathy (DR) can be well treated with early diagnosis. Retinal fundus images of humans are used to screen for lesions in the retina. However, detecting DR in the early stages is challenging due to the minimal symptoms. Furthermore, the occurrence of diseases linked to vascular anomalies brought on by DR aids in diagnosing the condition. Nevertheless, the resources required for manually identifying the lesions are high. Similarly, training for Convolutional Neural Networks (CNN) is more time-consuming. This proposed research aims to improve diabetic retinopathy diagnosis by developing an enhanced deep learning model (EDLM) for timely DR identification that is potentially more accurate than existing CNN-based models. The proposed model will detect various lesions from retinal images in the early stages. First, characteristics are retrieved from the retinal fundus picture and put into the EDLM for classification. For dimensionality reduction, EDLM is used. Additionally, the classification and feature extraction processes are optimized using the stochastic gradient descent (SGD) optimizer. The EDLM’s effectiveness is assessed on the KAGGLE dataset with 3459 retinal images, and results are compared over VGG16, VGG19, RESNET18, RESNET34, and RESNET50. Experimental results show that the EDLM achieves higher average sensitivity by 8.28% for VGG16, by 7.03% for VGG19, by 5.58% for ResNet18, by 4.26% for ResNet 34, and by 2.04% for ResNet 50, respectively.Keywords
Cite This Article
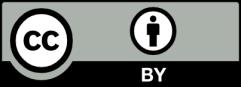
This work is licensed under a Creative Commons Attribution 4.0 International License , which permits unrestricted use, distribution, and reproduction in any medium, provided the original work is properly cited.