Open Access
ARTICLE
Bi-LSTM-Based Deep Stacked Sequence-to-Sequence Autoencoder for Forecasting Solar Irradiation and Wind Speed
1 Department of Electrical and Computer Engineering, COMSATS University Islamabad, Lahore Campus, 45550, Pakistan
2 School of Engineering and Technology, National Textile University, Faisalabad, 37610, Pakistan
3 Department of Electrical Engineering, Information Technology University, Lahore, 54000, Pakistan
4 Department of Electrical Engineering, GC University, Faisalabad, 38000, Pakistan
5 Faculty of Mechanical and Industrial Engineering, Warsaw University of Technology, 02-524, Warsaw, Poland
* Corresponding Author: Krzysztof Ejsmont. Email:
Computers, Materials & Continua 2023, 75(3), 6375-6393. https://doi.org/10.32604/cmc.2023.038564
Received 19 December 2022; Accepted 08 February 2023; Issue published 29 April 2023
Abstract
Wind and solar energy are two popular forms of renewable energy used in microgrids and facilitating the transition towards net-zero carbon emissions by 2050. However, they are exceedingly unpredictable since they rely highly on weather and atmospheric conditions. In microgrids, smart energy management systems, such as integrated demand response programs, are permanently established on a step-ahead basis, which means that accurate forecasting of wind speed and solar irradiance intervals is becoming increasingly crucial to the optimal operation and planning of microgrids. With this in mind, a novel “bidirectional long short-term memory network” (Bi-LSTM)-based, deep stacked, sequence-to-sequence autoencoder (S2SAE) forecasting model for predicting short-term solar irradiation and wind speed was developed and evaluated in MATLAB. To create a deep stacked S2SAE prediction model, a deep Bi-LSTM-based encoder and decoder are stacked on top of one another to reduce the dimension of the input sequence, extract its features, and then reconstruct it to produce the forecasts. Hyperparameters of the proposed deep stacked S2SAE forecasting model were optimized using the Bayesian optimization algorithm. Moreover, the forecasting performance of the proposed Bi-LSTM-based deep stacked S2SAE model was compared to three other deep, and shallow stacked S2SAEs, i.e., the LSTM-based deep stacked S2SAE model, gated recurrent unit-based deep stacked S2SAE model, and Bi-LSTM-based shallow stacked S2SAE model. All these models were also optimized and modeled in MATLAB. The results simulated based on actual data confirmed that the proposed model outperformed the alternatives by achieving an accuracy of up to 99.7%, which evidenced the high reliability of the proposed forecasting.Keywords
Cite This Article
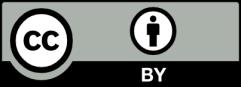
This work is licensed under a Creative Commons Attribution 4.0 International License , which permits unrestricted use, distribution, and reproduction in any medium, provided the original work is properly cited.