Open Access
ARTICLE
Fine-Grained Multivariate Time Series Anomaly Detection in IoT
1 School of Computer & Communication Engineering, Changsha University of Science & Technology, Changsha, 410114, China
2 Computer Science Department, Community College, King Saud University, Riyadh, 11437, Saudi Arabia
3 Department of Computing Science, University of Aberdeen, Aberdeen, AB24 3FX, UK
4 Hunan Provincial Key Laboratory of Network Investigational Technology, Hunan Police Academy, Changsha, 410138, China
* Corresponding Author: Xi’ai Yan. Email:
Computers, Materials & Continua 2023, 75(3), 5027-5047. https://doi.org/10.32604/cmc.2023.038551
Received 18 December 2022; Accepted 22 February 2023; Issue published 29 April 2023
Abstract
Sensors produce a large amount of multivariate time series data to record the states of Internet of Things (IoT) systems. Multivariate time series timestamp anomaly detection (TSAD) can identify timestamps of attacks and malfunctions. However, it is necessary to determine which sensor or indicator is abnormal to facilitate a more detailed diagnosis, a process referred to as fine-grained anomaly detection (FGAD). Although further FGAD can be extended based on TSAD methods, existing works do not provide a quantitative evaluation, and the performance is unknown. Therefore, to tackle the FGAD problem, this paper first verifies that the TSAD methods achieve low performance when applied to the FGAD task directly because of the excessive fusion of features and the ignoring of the relationship’s dynamic changes between indicators. Accordingly, this paper proposes a multivariate time series fine-grained anomaly detection (MFGAD) framework. To avoid excessive fusion of features, MFGAD constructs two sub-models to independently identify the abnormal timestamp and abnormal indicator instead of a single model and then combines the two kinds of abnormal results to detect the fine-grained anomaly. Based on this framework, an algorithm based on Graph Attention Neural Network (GAT) and Attention Convolutional Long-Short Term Memory (A-ConvLSTM) is proposed, in which GAT learns temporal features of multiple indicators to detect abnormal timestamps and A-ConvLSTM captures the dynamic relationship between indicators to identify abnormal indicators. Extensive simulations on a real-world dataset demonstrate that the proposed algorithm can achieve a higher F1 score and hit rate than the extension of existing TSAD methods with the benefit of two independent sub-models for timestamp and indicator detection.Keywords
Cite This Article
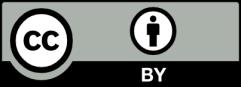