Open Access
ARTICLE
Visualization for Explanation of Deep Learning-Based Defect Detection Model Using Class Activation Map
1 Center for AI Technology in Construction, Hanyang University ERICA, Ansan, 15588, Korea
2 School of Architecture and Architectural Engineering, Hanyang University ERICA, Ansan, 15588, Korea
3 ICT Convergence Research Division, Korea Expressway Corporation Research Institute, Hwaseong, 18489, Korea
4 Department of Architecture, College of Engineering, Kangwon National University, Samcheok, 25913, Korea
5 Division of Smart Convergence Engineering, Hanyang University ERICA, Ansan, 15588, Korea
* Corresponding Authors: Jungsik Choi. Email: ; Sanghyo Lee. Email:
Computers, Materials & Continua 2023, 75(3), 4753-4766. https://doi.org/10.32604/cmc.2023.038362
Received 09 December 2022; Accepted 13 February 2023; Issue published 29 April 2023
Abstract
Recently, convolutional neural network (CNN)-based visual inspection has been developed to detect defects on building surfaces automatically. The CNN model demonstrates remarkable accuracy in image data analysis; however, the predicted results have uncertainty in providing accurate information to users because of the “black box” problem in the deep learning model. Therefore, this study proposes a visual explanation method to overcome the uncertainty limitation of CNN-based defect identification. The visual representative gradient-weights class activation mapping (Grad-CAM) method is adopted to provide visually explainable information. A visualizing evaluation index is proposed to quantitatively analyze visual representations; this index reflects a rough estimate of the concordance rate between the visualized heat map and intended defects. In addition, an ablation study, adopting three-branch combinations with the VGG16, is implemented to identify performance variations by visualizing predicted results. Experiments reveal that the proposed model, combined with hybrid pooling, batch normalization, and multi-attention modules, achieves the best performance with an accuracy of 97.77%, corresponding to an improvement of 2.49% compared with the baseline model. Consequently, this study demonstrates that reliable results from an automatic defect classification model can be provided to an inspector through the visual representation of the predicted results using CNN models.Keywords
Cite This Article
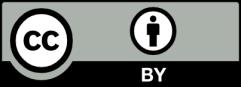
This work is licensed under a Creative Commons Attribution 4.0 International License , which permits unrestricted use, distribution, and reproduction in any medium, provided the original work is properly cited.