Open Access
ARTICLE
Multi-Attack Intrusion Detection System for Software-Defined Internet of Things Network
1 Pan African University Institute for Basic Sciences, Technology and Innovation, Nairobi, 6200-00200, Kenya
2 Department of Electrical and Control Engineering, Egerton University, Nakuru, 536-20115, Kenya
3 School of Engineering, American University of Nigeria, PMB 2250 Yola, Nigeria
* Corresponding Author: Tarcízio Ferrão. Email:
Computers, Materials & Continua 2023, 75(3), 4985-5007. https://doi.org/10.32604/cmc.2023.038276
Received 06 December 2022; Accepted 23 February 2023; Issue published 29 April 2023
Abstract
Currently, the Internet of Things (IoT) is revolutionizing communication technology by facilitating the sharing of information between different physical devices connected to a network. To improve control, customization, flexibility, and reduce network maintenance costs, a new Software-Defined Network (SDN) technology must be used in this infrastructure. Despite the various advantages of combining SDN and IoT, this environment is more vulnerable to various attacks due to the centralization of control. Most methods to ensure IoT security are designed to detect Distributed Denial-of-Service (DDoS) attacks, but they often lack mechanisms to mitigate their severity. This paper proposes a Multi-Attack Intrusion Detection System (MAIDS) for Software-Defined IoT Networks (SDN-IoT). The proposed scheme uses two machine-learning algorithms to improve detection efficiency and provide a mechanism to prevent false alarms. First, a comparative analysis of the most commonly used machine-learning algorithms to secure the SDN was performed on two datasets: the Network Security Laboratory Knowledge Discovery in Databases (NSL-KDD) and the Canadian Institute for Cybersecurity Intrusion Detection Systems (CICIDS2017), to select the most suitable algorithms for the proposed scheme and for securing SDN-IoT systems. The algorithms evaluated include Extreme Gradient Boosting (XGBoost), K-Nearest Neighbor (KNN), Random Forest (RF), Support Vector Machine (SVM), and Logistic Regression (LR). Second, an algorithm for selecting the best dataset for machine learning in Intrusion Detection Systems (IDS) was developed to enable effective comparison between the datasets used in the development of the security scheme. The results showed that XGBoost and RF are the best algorithms to ensure the security of SDN-IoT and to be applied in the proposed security system, with average accuracies of 99.88% and 99.89%, respectively. Furthermore, the proposed security scheme reduced the false alarm rate by 33.23%, which is a significant improvement over prevalent schemes. Finally, tests of the algorithm for dataset selection showed that the rates of false positives and false negatives were reduced when the XGBoost and RF algorithms were trained on the CICIDS2017 dataset, making it the best for IDS compared to the NSL-KDD dataset.Keywords
Cite This Article
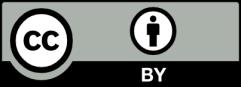