Open Access
ARTICLE
A Progressive Approach to Generic Object Detection: A Two-Stage Framework for Image Recognition
1 College of Computer Science, Huanggang Normal University, Huanggang, Hubei, 438000, China
2 FAST School of Computing, National University of Computer & Emerging Sciences (NUCES),
Karachi Campus, Karachi, 75030, Pakistan
3 School of Information and Communication Engineering, Hainan University, Haikou, 570228, China
* Corresponding Author: Ziaur Rahman. Email: ziaurrahman167@yahoo.com
* Corresponding Author: Ziaur Rahman. Email:
Computers, Materials & Continua 2023, 75(3), 6351-6373. https://doi.org/10.32604/cmc.2023.038173
Received 30 November 2022; Accepted 15 March 2023; Issue published 29 April 2023
Abstract
Object detection in images has been identified as a critical area of research in computer vision image processing. Research has developed several novel methods for determining an object’s location and category from an image. However, there is still room for improvement in terms of detection efficiency. This study aims to develop a technique for detecting objects in images. To enhance overall detection performance, we considered object detection a two-fold problem, including localization and classification. The proposed method generates class-independent, high-quality, and precise proposals using an agglomerative clustering technique. We then combine these proposals with the relevant input image to train our network on convolutional features. Next, a network refinement module decreases the quantity of generated proposals to produce fewer high-quality candidate proposals. Finally, revised candidate proposals are sent into the network’s detection process to determine the object type. The algorithm’s performance is evaluated using publicly available the PASCAL Visual Object Classes Challenge 2007 (VOC2007), VOC2012, and Microsoft Common Objects in Context (MS-COCO) datasets. Using only 100 proposals per image at intersection over union (IoU) = 0.5 and 0.7), the proposed method attains Detection Recall (DR) rates of (93.17% and 79.35%) and (69.4% and 58.35%), and Mean Average Best Overlap (MABO) values of (79.25% and 62.65%), for the VOC2007 and MS-COCO datasets, respectively. Besides, it achieves a Mean Average Precision (mAP) of (84.7% and 81.5%) on both VOC datasets. The experiment findings reveal that our method exceeds previous approaches in terms of overall detection performance, proving its effectiveness.Keywords
Cite This Article
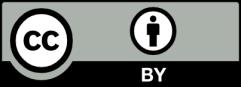
This work is licensed under a Creative Commons Attribution 4.0 International License , which permits unrestricted use, distribution, and reproduction in any medium, provided the original work is properly cited.