Open Access
ARTICLE
Research on PM2.5 Concentration Prediction Algorithm Based on Temporal and Spatial Features
School of Computer Science and Engineering, Central South University, Changsha, 410000, China
* Corresponding Author: Song Yu. Email:
Computers, Materials & Continua 2023, 75(3), 5555-5571. https://doi.org/10.32604/cmc.2023.038162
Received 29 November 2022; Accepted 15 March 2023; Issue published 29 April 2023
Abstract
PM2.5 has a non-negligible impact on visibility and air quality as an important component of haze and can affect cloud formation and rainfall and thus change the climate, and it is an evaluation indicator of air pollution level. Achieving PM2.5 concentration prediction based on relevant historical data mining can effectively improve air pollution forecasting ability and guide air pollution prevention and control. The past methods neglected the impact caused by PM2.5 flow between cities when analyzing the impact of inter-city PM2.5 concentrations, making it difficult to further improve the prediction accuracy. However, factors including geographical information such as altitude and distance and meteorological information such as wind speed and wind direction affect the flow of PM2.5 between cities, leading to the change of PM2.5 concentration in cities. So a PM2.5 directed flow graph is constructed in this paper. Geographic and meteorological data is introduced into the graph structure to simulate the spatial PM2.5 flow transmission relationship between cities. The introduction of meteorological factors like wind direction depicts the unequal flow relationship of PM2.5 between cities. Based on this, a PM2.5 concentration prediction method integrating spatial-temporal factors is proposed in this paper. A spatial feature extraction method based on weight aggregation graph attention network (WGAT) is proposed to extract the spatial correlation features of PM2.5 in the flow graph, and a multi-step PM2.5 prediction method based on attention gate control loop unit (AGRU) is proposed. The PM2.5 concentration prediction model WGAT-AGRU with fused spatiotemporal features is constructed by combining the two methods to achieve multi-step PM2.5 concentration prediction. Finally, accuracy and validity experiments are conducted on the KnowAir dataset, and the results show that the WGAT-AGRU model proposed in the paper has good performance in terms of prediction accuracy and validates the effectiveness of the model.Keywords
Cite This Article
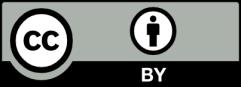
This work is licensed under a Creative Commons Attribution 4.0 International License , which permits unrestricted use, distribution, and reproduction in any medium, provided the original work is properly cited.