Open Access
ARTICLE
Multi-Attribute Couplings-Based Euclidean and Nominal Distances for Unlabeled Nominal Data
School of Computer, Nanjing University of Posts and Telecommunications, Nanjing, 210023, China
* Corresponding Author: Lei Gu. Email:
Computers, Materials & Continua 2023, 75(3), 5911-5928. https://doi.org/10.32604/cmc.2023.038127
Received 28 November 2022; Accepted 10 March 2023; Issue published 29 April 2023
Abstract
Learning unlabeled data is a significant challenge that needs to handle complicated relationships between nominal values and attributes. Increasingly, recent research on learning value relations within and between attributes has shown significant improvement in clustering and outlier detection, etc. However, typical existing work relies on learning pairwise value relations but weakens or overlooks the direct couplings between multiple attributes. This paper thus proposes two novel and flexible multi-attribute couplings-based distance (MCD) metrics, which learn the multi-attribute couplings and their strengths in nominal data based on information theories: self-information, entropy, and mutual information, for measuring both numerical and nominal distances. MCD enables the application of numerical and nominal clustering methods on nominal data and quantifies the influence of involving and filtering multi-attribute couplings on distance learning and clustering performance. Substantial experiments evidence the above conclusions on 15 data sets against seven state-of-the-art distance measures with various feature selection methods for both numerical and nominal clustering.Keywords
Cite This Article
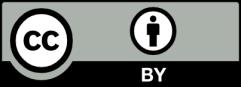