Open Access
ARTICLE
A Transfer Learning Approach Based on Ultrasound Images for Liver Cancer Detection
1 College of Computer and Information Sciences, Jouf University, Sakaka, 72314, Saudi Arabia
2 Department of Information Systems, MCI Academy, Cairo, Egypt
3 College of Medicine, Shaqra University, Shaqra, KSA
4 Faculty of Medicine, Al Azhar University, Cairo, Egypt
* Corresponding Authors: Ayman Mohamed Mostafa. Email: ; Mohamed Ezz. Email:
Computers, Materials & Continua 2023, 75(3), 5105-5121. https://doi.org/10.32604/cmc.2023.037728
Received 15 November 2022; Accepted 22 February 2023; Issue published 29 April 2023
Abstract
The convolutional neural network (CNN) is one of the main algorithms that is applied to deep transfer learning for classifying two essential types of liver lesions; Hemangioma and hepatocellular carcinoma (HCC). Ultrasound images, which are commonly available and have low cost and low risk compared to computerized tomography (CT) scan images, will be used as input for the model. A total of 350 ultrasound images belonging to 59 patients are used. The number of images with HCC is 202 and 148, respectively. These images were collected from ultrasound cases.info (28 Hemangiomas patients and 11 HCC patients), the department of radiology, the University of Washington (7 HCC patients), the Atlas of ultrasound Germany (3 HCC patients), and Radiopedia and others (10 HCC patients). The ultrasound images are divided into 225, 52, and 73 for training, validation, and testing. A data augmentation technique is used to enhance the validation performance. We proposed an approach based on ensembles of the best-selected deep transfer models from the on-the-shelf models: VGG16, VGG19, DenseNet, Inception, InceptionResNet, ResNet, and EfficientNet. After tuning both the feature extraction and the classification layers, the best models are selected. Validation accuracy is used for model tuning and selection. The accuracy, sensitivity, specificity and AUROC are used to evaluate the performance. The experiments are concluded in five stages. The first stage aims to evaluate the base model performance by training the on-the-shelf models. The best accuracy obtained in the first stage is 83.5%. In the second stage, we augmented the data and retrained the on-the-shelf models with the augmented data. The best accuracy we obtained in the second stage was 86.3%. In the third stage, we tuned the feature extraction layers of the on-the-shelf models. The best accuracy obtained in the third stage is 89%. In the fourth stage, we fine-tuned the classification layer and obtained an accuracy of 93% as the best accuracy. In the fifth stage, we applied the ensemble approach using the best three-performing models and obtained an accuracy, specificity, sensitivity, and AUROC of 94%, 93.7%, 95.1%, and 0.944, respectively.Keywords
Cite This Article
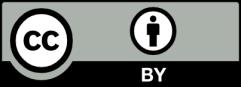