Open Access
ARTICLE
Improved Monarchy Butterfly Optimization Algorithm (IMBO): Intrusion Detection Using Mapreduce Framework Based Optimized ANU-Net
School of Computer Science and Engineering, VIT-AP University, Amaravathi, 522237, Andhra Pradesh, India
* Corresponding Author: Yamarthi Narasimha Rao. Email:
Computers, Materials & Continua 2023, 75(3), 5887-5909. https://doi.org/10.32604/cmc.2023.037486
Received 05 November 2022; Accepted 03 March 2023; Issue published 29 April 2023
Abstract
The demand for cybersecurity is rising recently due to the rapid improvement of network technologies. As a primary defense mechanism, an intrusion detection system (IDS) was anticipated to adapt and secure computing infrastructures from the constantly evolving, sophisticated threat landscape. Recently, various deep learning methods have been put forth; however, these methods struggle to recognize all forms of assaults, especially infrequent attacks, because of network traffic imbalances and a shortage of aberrant traffic samples for model training. This work introduces deep learning (DL) based Attention based Nested U-Net (ANU-Net) for intrusion detection to address these issues and enhance detection performance. For this IDS model, the first data preprocessing is carried out in three stages: duplication elimination, label transformation, and data normalization. Then the features are extracted and selected based on the Improved Flower Pollination Algorithm (IFPA). The Improved Monarchy Butterfly Optimization Algorithm (IMBO), a new metaheuristic, is used to modify the hyper-parameters in ANU-Net, effectively increasing the learning rate for spatial-temporal information and resolving the imbalance problem. Through the use of parallel programming, the MapReduce architecture reduces computation complexity while significantly accelerating processing. Three publicly available data sets were used to evaluate and test the approach. The investigational outcomes suggest that the proposed technique can more efficiently boost the performances of IDS under the scenario of unbalanced data. The proposed method achieves above 98% accuracy and classifies various attacks significantly well compared to other classifiers.Keywords
Cite This Article
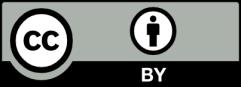
This work is licensed under a Creative Commons Attribution 4.0 International License , which permits unrestricted use, distribution, and reproduction in any medium, provided the original work is properly cited.