Open Access
ARTICLE
Deep Learning for Multivariate Prediction of Building Energy Performance of Residential Buildings
1 Department of ICT Convergence System Engineering, Chonnam National University, Gwangju, 61186, Korea
2 Graduate School of Data Science, Chonnam National University, Gwangju, 61186, Korea
3 Interdisciplinary Program of Digital Future Convergence Service, Chonnam National University, Gwangju, 61186, Korea
4 Department of Computer Engineering, Chonnam National University, Yeosu, 59626, Korea
* Corresponding Authors: Chang Gyoon Lim. Email: ; Jinsul Kim. Email:
Computers, Materials & Continua 2023, 75(3), 5947-5964. https://doi.org/10.32604/cmc.2023.037202
Received 27 October 2022; Accepted 15 January 2023; Issue published 29 April 2023
Abstract
In the quest to minimize energy waste, the energy performance of buildings (EPB) has been a focus because building appliances, such as heating, ventilation, and air conditioning, consume the highest energy. Therefore, effective design and planning for estimating heating load (HL) and cooling load (CL) for energy saving have become paramount. In this vein, efforts have been made to predict the HL and CL using a univariate approach. However, this approach necessitates two models for learning HL and CL, requiring more computational time. Moreover, the one-dimensional (1D) convolutional neural network (CNN) has gained popularity due to its nominal computational complexity, high performance, and low-cost hardware requirement. In this paper, we formulate the prediction as a multivariate regression problem in which the HL and CL are simultaneously predicted using the 1D CNN. Considering the building shape characteristics, one kernel size is adopted to create the receptive fields of the 1D CNN to extract the feature maps, a dense layer to interpret the maps, and an output layer with two neurons to predict the two real-valued responses, HL and CL. As the 1D data are not affected by excessive parameters, the pooling layer is not applied in this implementation. Besides, the use of pooling has been questioned by recent studies. The performance of the proposed model displays a comparative advantage over existing models in terms of the mean squared error (MSE). Thus, the proposed model is effective for EPB prediction because it reduces computational time and significantly lowers the MSE.Keywords
Cite This Article
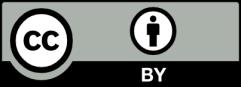
This work is licensed under a Creative Commons Attribution 4.0 International License , which permits unrestricted use, distribution, and reproduction in any medium, provided the original work is properly cited.