Open Access
ARTICLE
A Model for Helmet-Wearing Detection of Non-Motor Drivers Based on YOLOv5s
College of Computer & Information Engineering, Central South University of Forestry and Technology, Changsha, 410004, China
* Corresponding Author: Feng Jiang. Email:
Computers, Materials & Continua 2023, 75(3), 5321-5336. https://doi.org/10.32604/cmc.2023.036893
Received 15 October 2022; Accepted 08 February 2023; Issue published 29 April 2023
Abstract
Detecting non-motor drivers’ helmets has significant implications for traffic control. Currently, most helmet detection methods are susceptible to the complex background and need more accuracy and better robustness of small object detection, which are unsuitable for practical application scenarios. Therefore, this paper proposes a new helmet-wearing detection algorithm based on the You Only Look Once version 5 (YOLOv5). First, the Dilated convolution In Coordinate Attention (DICA) layer is added to the backbone network. DICA combines the coordinated attention mechanism with atrous convolution to replace the original convolution layer, which can increase the perceptual field of the network to get more contextual information. Also, it can reduce the network’s learning of unnecessary features in the background and get attention to small objects. Second, the Rebuild Bidirectional Feature Pyramid Network (Re-BiFPN) is used as a feature extraction network. Re-BiFPN uses cross-scale feature fusion to combine the semantic information features at the high level with the spatial information features at the bottom level, which facilitates the model to learn object features at different scales. Verified on the proposed “Helmet Wearing dataset for Non-motor Drivers (HWND),” the results show that the proposed model is superior to the current detection algorithms, with the mean average precision (mAP) of 94.3% under complex background.Keywords
Cite This Article
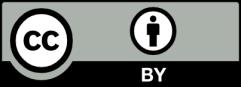